Note: This is Part 4 in a short series of essays on Understanding Customer Demand. Read Part 3 here.
Let me tell you a startup horror story. If you’ve been around startups for long enough, you’re likely to recognise this narrative. You may have — like me — lived something like it before.
I want you to imagine that you are a founder of a startup. You make cybersecurity software that detects and blocks botnet attacks. This is 2006, so you are very early to this market — so early, in fact, that the name ‘botnet’ isn’t actually a thing yet. Nobody knows what to call them, so your lab mates call this threat pattern ‘bot armies’.
You are associated with a cybersecurity lab at Georgia Tech. You’ve been monitoring the growth of this threat for a while now. The lab has developed some cutting edge technology to deal with botnets, and you think you might be on to something big. One day, you show it to the Chief Information Security Officer of eBay, a multi-billion dollar company, and he asks you to come to their offices for a presentation. You do so. eBay’s security team does some back of the envelope math after your presentation, in front of you, and they tell you that they suspect they’re losing $40 million a year in botnet enabled fraud. They ask you how much you’re willing to charge for your solution. You pull a number out of your ass and say “$150,000 a year, to start.” They say: “how soon can you deliver?”
This is the point where you start to get very excited.
I’m going to present you with a number of other facts about your company, and then I’m going to ask you three questions. So, let’s get this straight:
- eBay tells you that they want to buy your solution. Even better, eBay CISO and cybersecurity legend Howard Schmidt tells you that they are losing $40 million dollars a year to botnet-enabled fraud. They make it sound like $150k is nothing in the face of such a threat.
- Many similarly sized companies tell you that they want to buy, at the same level of eagerness as eBay.
- Even before you start your company, a security company pays you $100k a year for access to a data feed produced by your technology. This is actual money! Paid to you!
- By some calculations, botnets are lodged in 17% of all computers in the world circa 2006, and this is only going to get worse. This is a massive, looming problem, and everyone that runs a business on the Internet is going to be affected. You bet that increasing numbers of people are going to realise this soon. The ‘total addressable market’ (or TAM), as they say in business-land, is going to be huge.
- You are also one of the first teams with cutting-edge, proprietary technology for this threat; over the next few years, you are one of only a few companies playing in the space.
- You negotiate the IP from Georgia Tech, form a company, and raise a round from VCs in a very short amount of time.
So here are my questions:
- First, do you think this company has — at this point — discovered demand?
- Second, do you think this company will find product market fit?
- Third, do you think this company will result in a venture-scale outcome?
If you’re anything like me, you would say “yes” to all three questions, though perhaps with a ‘maybe’ slant to the third. I mean, demand at this sort of clarity and strength is remarkably rare. People were paying money even before the company was formed, for goodness sakes!
But, alas, the answer to all three questions is “no”:
- No, they had not discovered demand.
- No, they never found product market fit for the entire duration of the startup’s life.
- No, the company burnt $69 million dollars and was sold for $9 million a decade later — which was a loss for all the investors involved, save the ones who participated in the final round.
The name of this company was Damballa. It was founded by Merrick Furst and Matt Chanoff in 2006. It was sold for parts in 2016. We document the full case below, and I recommend reading the complete thing to internalise how tricky demand can be. Of course, if you’ve worked with startups or spent any amount of time in startup land, you won’t be unduly surprised. Such things do happen. Here’s an excerpt, to give you an idea of how difficult it was:
More than a decade later, Furst and Chanoff would reflect on what happened next:
Everyone at Damballa believed that all the elements that made up demand were in place. The product would save customers a large amount of money, it worked the way they needed it to, and we had a competent team and sufficient capital to operate. Most important, we all had a fixed idea in our heads that we never questioned: companies would not tolerate their machines being compromised. Bots hiding secretly on the company computers led to all sorts of risks. Click fraud, trust fraud, stealing passwords, eavesdropping on company emails, stealing proprietary data or customer information—we made up examples, and we heard examples from people like Howard. We didn’t feel stuck. Even as sales lagged expectations, we always felt that we saw the problem and could move forward by fixing it. Maybe our software increased processing time. Maybe putting third-party software like ours inside customers’ firewalls was too risky for them. Maybe putting it outside their firewalls made them feel vulnerable. Maybe the particular examples of bad things bots could do weren’t hitting home and we had to change the marketing.
These were all obvious, reasonable actions. The team found a replacement CEO and Furst moved himself to the board. Over the next several years, as sales to other companies fell well short of expectations, internal company and board conversations kept revolving around the same issues. They did everything a company on the cusp of a major breakthrough would do: they replaced key management personnel, improved the product, raised more money — repeatedly, over many years, eventually deploying $69 million in venture capital. The technology was novel, the target market was large and lucrative … what was wrong?!
As management and the board worked to get the company on track, they addressed all the conventional issues. They believed in a very clear idea of why there should be demand, and worked on the basis of that belief. They thought the customers were compromised by bots and that they would buy things to fix that—that they would not not buy because they couldn’t allow themselves to be compromised. That view stayed at the root of all the company’s plans and tactics, and it didn’t budge. Internally, there were variations on that basic belief. Some people thought that money was the issue: customers would buy because being compromised cost them money. Others thought our customers would be afraid that their customers would be scared away or displaced by bots. Still others thought risk was the issue: customers would buy because they were otherwise vulnerable to fraud allegations.
With hindsight we can see that these just aren’t effective ways to understand customer demand. The right question ought to have been, “What ever gave us the impression that eBay would be a customer?” On what basis did we believe that our preferred value proposition would actually drive sales? (emphasis added)
Furst would later describe those years as “living in a waking dream.” In The Heart of Innovation, his 2023 book with the answers to many of these questions, Furst wrote that conversations during this period was extremely painful, because everyone felt like they knew what they were talking about and yet nothing they said had reliable predictive power. Even when they disagreed, their core premises were all the same: “Customers bought due to a value proposition. They bought based on certain properties that they possessed, and that the product possessed.”
But Damballa’s value proposition was perfect. And yet it did not lead to sustained demand. Furst observed that such logic didn’t work — at least not universally. Which meant that it wasn’t very useful.
At this point I should remind you that 10 years is a long time. Most careers last 40 years. 10 years is a quarter of that. Burning a quarter of your career on a startup with no real demand is a huge hit to take. I want you to really imagine this. If you start a company in your 20s, by the time you’re done, you’re in your 30s and you are married and are thinking about having kids (assuming you want them, and if you don’t have them already) … that, plus your parents are getting old. If you start a company in your 30s, by the time you’re done, you’re in your 40s, and if you have kids they take up a lot of your time and your parents are having serious health issues. Most people don’t have the same levels of energy in their 40s that they do in their 20s. And it’s not even that easy to tell that you are stuck in a dead end startup — as the Damballa case demonstrates, it is absolutely possible to be burning through all those years believing that you’re on the cusp of a breakthrough, that you just have to make the right set of small modifications to turn the trickle of sales into a torrent, but you’re losing money as you’re figuring all of this out and in truth you actually have no hope.
This is why we need a better theory of demand. A quarter of your career is a very high cost to pay. You can always make more money, but you aren’t ever going to get that time back.
In the previous instalment I presented a demand-side mystery using the index fund manager, Vanguard, as an example. In the newsletter for that piece I argued that if your demand-side framework cannot explain Vanguard — a 10 trillion dollar asset manager — then it probably isn’t very good. More importantly, I explained the history of demand frameworks — that pretty much all of them are built around the idea of pain (or the flip side of pain: desire). I explained that defining demand as about pain is problematic — it doesn’t explain the full set of successful companies, and you cannot reliably use pain as a positive signal for new product creation.
Well, the good news is that the founders of Damballa spent the subsequent years puzzling over their decade-long experience. The result of their thinking and then experimentation was — first, Flashpoint, an incubator program at Georgia Tech with a remarkable track record. (We’ll talk about that track record in a bit). And, second, a set of ideas around demand that I believe are the first major contribution to the state of the art since the Jobs to be Done framework. These ideas were published as the book The Heart of Innovation in 2023.
Furst’s and Chanoff’s ideas happen to be the first thing I’ve seen that helps explain Vanguard. They call their approach ‘Deliberate Innovation’. The goal of Deliberate Innovation is to identify ‘authentic demand’ — that is, the kind of demand that means product-market fit, instead of the fake demand Furst and Chanoff suffered through in Damballa. These ideas were developed with the goal of preventing a founder from falling into a similar, Damballa-like trap. They paid the price so that we might do better.
Let’s get into it.
Situations, not Psychographics
The first big idea in Deliberate Innovation is about situations.
There’s this old, famous story about Ignaz Semmelweis, the Hungarian doctor who, in 1846, discovered that washing your hands with chlorine right before delivering babies would reduce the rate of childbed fever — a common disease that, at the time, killed many mothers shortly after childbirth.
Semmelweis’s story is usually told as a cautionary tale: he was initially successful at pushing this change, but was such an abrasive character that eventually doctors stopped listening to him, influential figures at his hospital got him fired, and Semmelweis found himself in career purgatory until he broke down and was admitted to a mental asylum when he was 47 years old. Semmelweis died just a few years later.
Semmelweis’s story is a tragedy. But there’s also a way to read this as a story of demand. Two decades after Semmelweis passed, germ theory beat out the miasma theory as the prevailing theory of disease. As the theory took hold, it became unacceptable for doctors to not wash their hands before treating patients. You could say that demand for soaps and other disinfectants grew as the germ theory of disease took hold.
What changed? The answer is not customer pain, nor customer desire. The idea that doctors suddenly desired soap is not the right frame to use. (Even the notion that “doctors use soap to avoid the pain of killing patients” or “the value proposition of soap is to ensure you don’t kill your patients” is also not accurate — you are trying to explain consumer behaviour through the underpowered concept of pain.) What changed was simply … default behaviour. After germ theory won, no surgeon or doctor could not not wash their hands before treating a patient. It would be unconscionable. It would be disgusting. It was just something that no doctor would ever do.
Embedded in that last sentence lies the solution to our puzzle.
In human behaviour, there are actually many things that we do that do not readily fit into models of desire, or pain avoidance, or progress. This is an old idea from psychology. Sometimes what we do depends not on what traits we have, but what situations demand of us. The most extreme, infamous demonstration of this hypothesis is probably Phillip Zimbardo’s 1971 Stanford Prison Experiment, in which students were divided into prisoners and guards, and then guard behaviour became so abusive and so cruel that the experiment had to be stopped early. (There are many problems with this experiment, but it continues to be cited when talking about the ‘situationist’ theory of human behaviour.)
But this isn’t just theoretical. Situation design is also useful in many real-world environments. Take, for instance, how a stage magician gets an audience member to participate in a magic trick. If the magician points at a random person and says “come up here and pick a card”, there is a possibility that the pick fails. The audience member might shake her head, refusing the offer, forcing the magician to try again with another person. This can rapidly gum up an act.
So this is not what magicians do. In order to guarantee audience participation, what a stage magician typically does is something like this:
- Point to a member of the audience and ask “ma’am, what is your name?”
- When the person answers “Jane”, the magician goes, “Jane, would you please stand up?”
- As Jane stands up, the magician goes: “Everyone, give Jane a round of applause!”
- And then after the applause dies down, the magician says “Jane, would you do me a favour: come up here and pick a card.”
With this chain of events, the magician creates a series of situations where the audience member cannot not do what the magician wants her to do. In fact, it doesn’t matter if the person is shy or if they would not normally stand up in front of a crowd — the situation overrides their personality.
And so it is with sales. To be fair, good sales and marketing leaders already recognise this. Most good sales leads I know may talk about traits, like the firmographics or psychographics of an Ideal Customer Profile, but if you watch them in action you will notice they naturally also think about the situation they are selling into. This makes sense: humans are pattern matching machines, and good go-to-market folk naturally pattern match across successful deals. It is inevitable that situations will come up as part of the success pattern.
So the first big idea in The Heart of Innovation is that situations matter as much if not more than psychographics or demographics when evaluating demand. Another way of putting this is that people buy based on the situation they find themselves in, not just based on who they are or how they see themselves. The straightforward application of this is that if you’re doing sales, you should study the situations that people buy, and then find a way to hunt down or create more such situations. But this is not a novel idea. And — even if it was — existing frameworks already exist that help you when you’re identifying demand in the context of a sales motion.
Where Deliberate Innovation shines is that it extends this idea to the earliest stages of product creation. If you’re creating a new product, one that has never been sold before, then the recommendation is slightly different: demand hides in the situation. Furst and Chanoff teach founders to study situations in order to identify demand. We’ll talk about how they do that in a bit, but first we need to talk about one final, major idea that is actually the primary contribution of The Heart of Innovation.
The Not Not
We’ve just seen that Deliberate Innovation’s first idea is about situations: that situations can be as if not more important than customer traits when searching for demand. Deliberate Innovation’s second idea is a razor that builds on this: a precisely crafted notion of what demand looks like. This idea is actually the more important one, because the ability to identify authentic demand tells you when you don’t have it. This allows you to avoid a Damballa-like outcome.
The razor is this:
Authentic demand exists for a solution when someone is put in a situation and they cannot not buy (or use) the solution.
The authors call this the ‘not not’, and may be used in a sentence like so: “the job of the founder is to find a ‘not not’.”
Why is this frame superior to the traditional method of evaluating demand?
The key lesson that Furst and Chanoff took from their Damballa experience is that evaluating demand in terms of value propositions, desires or pains is simply not predictive. Damballa had the ‘perfect’ value proposition: it had eager customers, a massive and ever-increasing pain, and existing commitments before company formation. If it was presented to you as an investor, back in 2006, you’d say “where can I invest?” … and then you’d wait 10 years and lose all your money.
In truth, the following points are all observably true:
- Pain is not predictive because you and I have many pains that we don’t do anything about. We learn to cope. And so if we do not act on all our pains, then interviewing for pain is actually a crapshoot. There is no way to know, ex-ante, that a person or business’s pain point is something that you can build a product around. The only way to know is to build the product first and attempt to sell it later — a highly risky affair.
- Desire is not predictive for the same reason. You want lots of things but you only buy some of the things that you desire. So interviewing for desire doesn’t guarantee anything!
- Innovation frameworks like ‘Lean Startup’ and the ‘Customer Development Model’ do not work reliably.
- And in fact, all the existing demand frameworks (including the two that we’ve studied) assume that a purchase decision has already been made before you interview for pain. This is an entirely different situation from the one you find yourself in at the earliest stages of new product development. If a purchase has already occurred, demand may exist; you may work backwards to find pain that is be a good predictor of future purchases. But you cannot take a random pain and work forwards with any guarantee that it would result in authentic demand.
- The obvious conclusion is that authentic demand can exist whether or not pain exists. The two are independent things. The proof of this is that there are companies that have tapped into authentic demand that do not solve any explicit pain. Facebook, Tiktok, and Instagram are good examples of this. For these companies, what you will find is that users with access to these apps cannot not reach for them when put in many, many situations. They have become habituated. Vanguard is also a good example of the same phenomenon, albeit with different dynamics. In all of these companies, it is more accurate to say that default behaviour changed in relation to a product. This is a more precise way of describing consumer behaviour, as opposed to shoe-horning that change into the language of pain or desire. (In the latter example, Vanguard succeeded because the consensus view on investing for retirement changed, and Vanguard benefited because it was waiting, perfectly positioned to receive that torrent of demand. We’ll talk more about Vanguard in a bit.)
- Finally, decoupling demand from pain gives you more tools in your toolbox. For instance: demand can be influenced if you change the situation. Here’s an example: if you make statistical software, and you get the US Food and Drug Administration (FDA) to mandate that all new drug applications must be submitted in a proprietary file format produced by your statistical software, then guess what: you may not have solved a pain or satisfied a desire any better than you already were, but you have created everlasting demand. This is the basis of SAS Institute’s moat, which we’ve discussed in the past.
Demand is tricky! Anyone who has spent time building new products or startups feels the slipperiness of demand in their bones. This is why Eric Ries’s Lean Startup, and Steve Blank’s Four Steps to the Epiphany do not work consistently. This is why I argued that the Idea Maze is actually a useless idea, and why expertise research into entrepreneurs finds that repeat founders accept the skill of entrepreneurship as one of improvisation. Identifying and pinning down demand is the central problem of all new venture creation.
The ‘not not’ is the first attempt I’ve seen to conceptualise demand without resorting to the language of pain — the predominant model of demand since Theodore Levitt’s 1983 book The Marketing Imagination. And it actually accomplishes a lot by doing so.
First, notice the double negative. The ‘not not’ is incoherent to think about — and this is by design. Why? Well, the biggest problem that founders have when validating their startup idea is that they are biased: they want their ideas to work. It is too easy to come up with a coherent story around a value proposition. In the process, founders trick themselves, and then their investors, and perpetuate a lie that can go on for years. Framing authentic demand in terms of a ‘not not’ makes reasoning about demand slightly more incoherent, and therefore makes it marginally harder to perform this self deception.
Second, notice how the ‘not not’ formulation removes certain avenues you may use to lie to yourself. When demand is expressed in terms of ‘value propositions’, you might think: “ahh, we are at the cusp of demand — people tell us they love our product, they love our idea, this problem costs them $40 million a month, but we just need to fix A, B, C in order to get them to buy.” This may sometimes be true — as in, for some products in some situations you might fix A, B, and C and hit the jackpot, but it is not consistently true, and so it is too easy to abuse. Instead, using the ‘not not’ razor is more brutal: “nope, we haven’t found a situation in which people cannot not buy our solution. We think we should modify A, B, or C and try again. Should we give it another go? Or should we give up?” The first framing allows you to do the sleight of hand thing where you assume demand currently exists. The latter framing removes that option. It forces you to reckon with the possibility that no demand might be present, and that you are wasting your time. It changes the tenor of the discussion.
A Better Definition of Product Market Fit
The real power of the ‘not not’ is that it gives us a better, more precise definition of product market fit (PMF).
For years now, experienced founders and VCs have attempted to describe PMF in a variety of ways:
- “You know it when you see it” is something that you hear from folks who have seen PMF happen at least once.
- “In a great market — a market with lots of real potential customers — the market pulls product out of the startup” — this one is from Marc Andreesen, who wrote one of best early attempts at describing PMF. In the same piece, he writes: “You can always feel product/market fit when it’s happening. The customers are buying the product just as fast as you can make it — or usage is growing just as fast as you can add more servers. Money from customers is piling up in your company checking account. You’re hiring sales and customer support staff as fast as you can. Reporters are calling because they’ve heard about your hot new thing and they want to talk to you about it.”
- Sequoia uses the analogy of the ‘head on fire’. To cite Michael Seibel, talking about Sequoia’s analogy in the context of PMF: “If your friend was standing next to you and their hair was on fire, that fire would be the only thing they really cared about in this world. It wouldn’t matter if they were hungry, just suffered a bad breakup, or were running late to a meeting—they’d prioritise putting the fire out.”
The problem with all of these definitions is that they are imprecise. I am absolutely certain that experienced VCs and founders are able to identify PMF when they see it, but coming up with a universal razor that captures their felt sense is a lot harder than it seems. You can read a particularly noteworthy approach by serial entrepreneur Jason Cohen here — he attempts to document what PMF looks like by pairing anecdotes with observable data.
But the not not gives us a better way of defining PMF. Put simply, you have PMF when you’ve found authentic demand. As a reminder, authentic demand for a solution is defined as: when someone is put in a situation, they cannot not buy (or use) the solution.
Why is this useful?
To founders, this is obvious: it is useful because it gives you clear guidance when you’ve found PMF. It’s not particularly useful to have an advisor tell you: “you want to be in a head-on-fire situation” or “you’ll know it when you see it.” It’s even less useful when the advisor extends that advice to “you need to find a painkiller.” It is much easier to ask: “is our prospective customer ok with not buying our solution?”. If the answer is no, you have PMF (contingent on finding more customers in situations like this); if the answer is yes, you do not.
For VCs, defining PMF in this way means that you have a method of picking startups who have found PMF just a bit before the adoption curve becomes clear to other investors. If most VCs have to ‘I know it when I see it’ — they would have to wait a bit for the adoption curve to become clear. But if you have the clarity of the ‘not not’ razor, you could verify PMF qualitatively — months before signs of PMF shows up in a startup’s adoption curve. Of course, I am aware that this is a limited advantage: if this essay is successful, it will result in the idea being more broadly known, meaning that more VCs are able to identify PMF at earlier stages. Already, in the Commoncog forums, a tiny handful — most notably James Cham of Bloomberg Beta — have picked up on the value of this razor.
This is, incidentally, why Furst’s and Chanoff’s Flashpoint incubator was so successful. I am not allowed to talk about their returns, but suffice to say I am quite impressed with their track record. The bit that I am allowed to talk about is that Flashpoint’s kill rate was extremely high. This meant that Furst and Chanoff were particularly good at killing startups that had not found authentic demand by the end of the program; as a result, Flashpoint’s hit-rate as percentage of cohort was far higher than pretty much any other incubator. And it’s not difficult to see why: they simply did not make follow-on investments in startups that hadn’t found authentic demand. It is trivially easy to do so when you have such a clear razor.
(This bit of Flashpoint’s record is also, I speculate, a result of their experiences — I’m willing to bet they wanted to spare others from the experience they had with Damballa. I should also note that Furst and Chanoff are now semi-retired: both have had remarkable careers and have no need to continue running an incubator. Flashpoint no longer exists. Their ideas are now being carried forward through a successor program run by Erik Reinertsen and partners.)
I have presented authentic demand as a superior definition of product market fit. But it might actually be better to drop the use of the phrase ‘product market fit’ whilst you are actively searching for it. PMF describes a desirable end state, but is not precise enough to identify potential problems during the search for that state, or even potential problems that may occur shortly after finding the state. Instead, I recommend adopting the language of ‘authentic demand’, ‘not nots’ and ‘situations’. This gives us better language to describe a variety of phenomena that you often see in startups. So, for instance:
- Startup veterans know that it is possible to lose product market fit. As an example, startups that provided virtual event software like Hopin and Welcome exploded with demand during the Covid pandemic, as a result of lockdowns. They all lost PMF and died after Covid ended. Talking about these companies with the language of ‘gaining’ or ‘losing’ PMF is not particularly useful, because it makes it difficult to tell when you are starting to lose it. With the language of authentic demand, though, it is easier to reason about when and how authentic demand can disappear. What happened was this: many companies had large events budgets that couldn’t be used during the Covid lockdowns; when put in this situation they couldn’t not spend that budget because they would lose the money if they didn’t. And so, all of that cash went to polished virtual events products from Hopin, Welcome, and — to a lesser extent — Zoom. After the pandemic ended, the situation that created this not not reverted. Companies redirected spend back to in-person events, and all of these startups saw their authentic demand vanish. The language of authentic demand is more descriptive, and therefore more actionable: you would be primed to look for changes in situations (and changes in not nots) as the pandemic wound itself down.
- It is also possible to have found a ‘not not’ for a particular situation, only to discover that there is no easy way to find similar situations. Having this language differentiates between the intensity of demand, and the actual addressable demand in a market. It also narrows the problem during a search for demand: is there a way to reliably create or reach customers in such situations?
- Finally, using the language of authentic demand allows us to identify when demand emerges over a long period of time. This is something that existing PMF discourse cannot handle. In the case of Vanguard, it took 15 years for index funds to reach large scale adoption. But authentic demand did exist in the early days, especially after John Bogle got rid of their load fees in 1981. In fact, this was likely the turning point: SEC approval for this bolstered the low fee argument for passive investing and paved the way for more institutional investors to reconsider index funds as a viable allocation option. Eventually, as the evidence against active management performance and fees grew, more and more investors could not not allocate to index funds.
Of course, the upshot of all this is that authentic demand is still a bit of a negative art. Having the concept of authentic demand doesn’t actually help you find it; the search is still idiosyncratic, as I’ve argued in the past. But it gives you two powerful things:
- It tells you when you’ve found it.
- And it prevents you from lying to yourself.
Solving the Vanguard Mystery
Let’s finally talk about the Vanguard mystery that I presented in the previous instalment of this series.
The short version of how Vanguard won was that default behaviour changed in the 15 years after the launch of their first index fund. Like the example of the doctors and germ theory earlier, the indexing argument gained ground, inexorably, over the course of decades. Today, nobody denies the merits of passive investing; the evidence is too great.
I think there are two questions that you should ask when faced with the Vanguard case. First, was it possible to predict the eventual dominance of passive investing over active investing? There is no law of nature to say that passive should have done so well. Perhaps passive would only gain so much ground, and active investing would continue to dominate. Second (and this question is the more important one for founders): was it possible to identify authentic demand early in Vanguard’s life?
The answer to the first question is, I think, no. I do not believe it was possible to predict the absolute dominance of passive investing today. Passive’s growth took even Vanguard founder John Bogle by surprise. But on the second question, I think that: yes, it was entirely possible. That is: I think that if you were equipped with the ideas of Deliberate Innovation back in the 70s, it would’ve been possible to identify demand relatively early in Vanguard’s life. Possibly by the late 70s, but definitely by the early 80s.
One reason I believe this was that there were already situations in which index funds won, even before Vanguard had launched. But you would have to look carefully. An early one came from the pension funds of the Baby Bells — the regional companies that were the result of a breaking up of the AT&T telephone company. Before the breakup, the regional Bells each had their own pension plans, and the managers of these plans had started looking into indexing by the early 70s. In Trillions, Robin Wigglesworth’s book about the rise of passive investing, the contributions of these managers are described thus:
However, the most important direct boost came from the so-called Baby Bells. (…) They became early and important adopters of index investing, after studying how their swelling investment plans were faring in the early 1970s. “They found out that their active managers were basically swapping bananas. One part of the system would be selling IBM stock, and another part would be buying IBM stock at the same time.” [manager of early WFIA S&P 500 index fund Thomas] Loeb says. “Their simple deduction was that they could cut down costs by indexing.”
Moreover, some pension executives began to slowly realize that many of the fund managers had hired were in reality little more than “closet indexers.” In other words, they essentially just mimicked the performance of the stock market as a whole, but charged fees as if they were engaged in an expensive hunt for the best securities. That spurred many to shift their allocations. “We figured we should pay index fees for index management,” George Williams, the head of Illinois Bell, told the Wall Street Journal in 1979. He became a particularly sizable backer of the first batch of index funds.
By the end of 1977, there was about $2.9 billion of pension fund money in the smattering of index strategies that had been launched. The 1974 bear market was a big impetus, but the longer-term picture slowly becoming clearer at the time was also grim. AG Becker, a prominent finance industry consultancy, found that 77% of US pension managers had trailed behind the S&P 500 in the decade ending December 1974. As a result, the amount of pension money to index funds, or internally managed [indexing] strategies, soared to about $91 billion by 1985, with a flurry of big new entrants into the nascent index fund industry, such as State Street and Bankers Trust.
When I told Furst about this story, I asked him if we could be more concrete about the types of not nots he’d seen. He told me that there were a few that came up repeatedly:
- Physical or logical impossibility (think about attempting to submit to the FDA clinical trial data in a format other than the mandated standard).
- Social unacceptability
- Value system violation
- Habituation (perhaps due to exposure to a secondary reinforcer)
- … and psychological paralysis (if consumers find alternative actions uncomfortable or incoherent, they would take a ‘default’, psychologically comfortable path).
We can do a slightly better job of explaining Vanguard’s success through this language: in the initial years, indexing’s adoption resulted from a value systems violation. Pension fund managers noticed that many of the actively-managed funds they invested in delivered a subpar return for excess fees. Exposed to this knowledge (and with more potent arguments slowly trickling out from academia), they could not not switch certain allocations to passive, because they acted as fiduciaries for their pensioners. As time passed, and as passive investing became the norm rather than the exception, passive investing began to benefit from social unacceptability. The arguments in support of passive and the influence of their institutional promoters titrated down to groups of retirement advisors, and then personal finance writers who published books on the topic, and eventually financial influencers on the Internet. Retirement savings had to be invested somewhere, and as active investing of various types became more and more socially unacceptable to retail, all of that money started going to index funds.
Furst warned me that it was not necessary to categorise the not nots. As a founder, you care that a not not exists, not that a not not ‘belongs’ to one category or another. “There is an old way of thinking about behaviours,” he told me, “which is that behaviours are always going to be things that people are impelled towards or restrained from.” The point: it is more productive to just reason about the sorts of behaviours that emerges in a particular situation. What pushes people towards or away from certain behaviours? Why? A typology does not help you much with this approach; being curious about the situation is more important.
Furst also said that he didn’t like this sort of slow-growing demand — at least for venture-backed startups. “You don’t know when it’s going to tip over,” he said, “And you don’t know if you’re going to be the winner when it finally tips over.” He’s right, of course: if you’re making a venture-style bet, you have to generate a return in a relatively short amount of time — 10-15 years, at most. The venture model is not well-positioned for long-term compounding bets. Thankfully, John Bogle had no such constraints.
How To Find Authentic Demand?
How do the authors recommend that we use their ideas? What methods do they teach their startups?
At this point, I’d like to issue a warning. Those of you who have read Commoncog for some time would know that I have a track record of taking ideas I’ve read and putting them to practice. I spent eight months to get Amazon’s PR/FAQ format to work for me, for instance. I’ve put Applied Cognitive Task Analysis to practice — a stripped down version of tacit knowledge extraction, though I still struggled with the format. Over the course of 2023 and 2024 I figured out how to apply the Amazon Weekly Business Review to my business. More recently, I executed a Jobs-to-be-Done interviewing project, which took about four months from start to finish; the first month of which was a series of terrible, horrible mistakes. I did eventually get it to work, though, which is a testament to the quality of written instructions available for the framework. In some of these cases I managed to get the methods to work by sheer trial and error; in other cases I had to get help from folks who were there when the method was invented.
So please believe me when I tell you that the process Furst and Chanoff describes in their book is impossible to put to practice from just reading alone. This is the sort of thing that will require apprenticeship from someone who has done it before, or at least a year of serious trial and error. During my conversation with Furst, Erik Reinertsen — who now runs the successor program to Flashpoint and was also present for the call — assured me that it took him a long time to grok as well. I do not believe that you (or I) can execute the framework that Furst and Chanoff presents in the book — at least not without serious guidance — so I’m only going to give you a basic sketch. If you want to seriously engage with these methods, I recommend reaching out to the authors, attending one of their workshops (if available), or buying the book to read carefully — with the expectation that you still have to do a year’s worth of trial and error.
That said, I think there are three, easy-to-use ideas here:
- Furst told me that if nothing else, one thing you can put to practice immediately is to ask your prospective customers: “Hey, I’m thinking of making this thing. Would it be ok if I don’t make it? Would it be possible for you not to buy?” This is a big upgrade over “would you be willing to buy?”-type conversations. In practice, though, Furst warned me that most founders would not be able to ask this — what will come out of their mouths is “will you buy it?”
- One of the methods that Furst and Chanoff teaches is a thing called a ‘situation diagram’. I believe this diagram can be put to practice easily, and may be adapted for other go-to-market research motions.
- I think the basic ideas of Deliberate Innovation — as presented in this essay — are potent enough that you could probably integrate them into your own work. This is especially true if you currently have experience with demand. If you do, it shouldn’t be too hard to come up with your own unique methods; the razor by itself is a huge help.
But let’s get down to it.
Situation Diagrams
The first tool that the authors created is a thing called a Situation Diagram.
When you're hunting for demand, you need a way to document the situations that you find. These include situations that your prospective customers are in, but also situations that include other stakeholders, like suppliers or perhaps your customer’s customers. How do you record this? Well, you record it using a Situation Diagram.
A Situation Diagram looks like this:
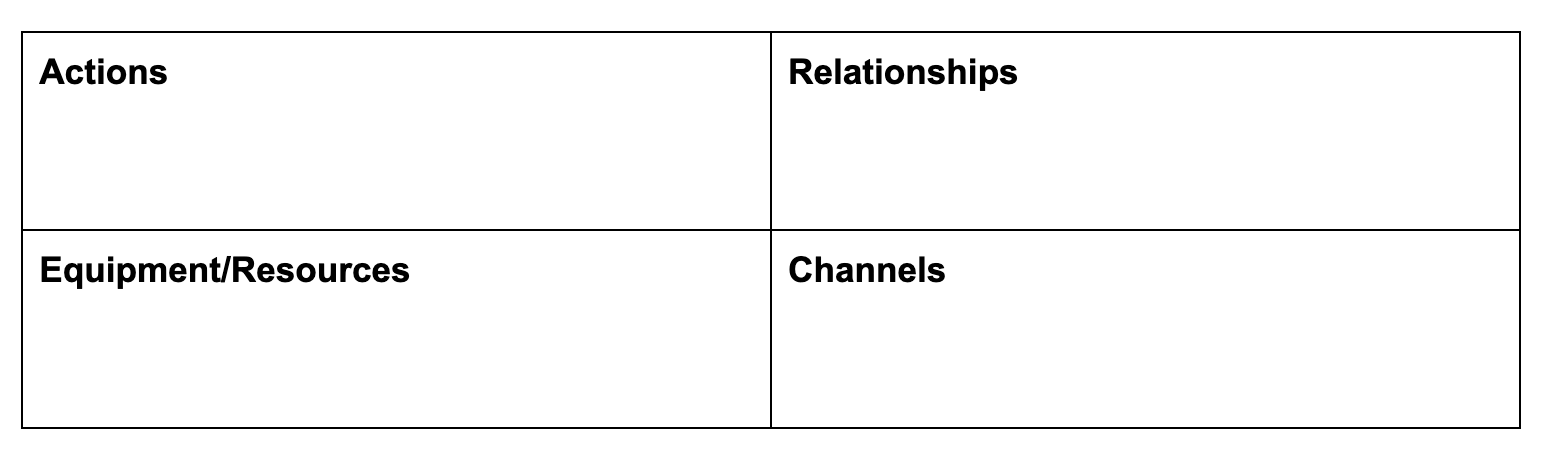
The four boxes represent the four parts of a situation that an observer can see and test:
- Actions: people doing things.
- Equipment/Resources: products and services used in the performance of actions.
- Relationships: activities by people other than the person whose actions we’re looking at, serving as a resource for those actions
- Channels: pathways by which equipment or other people’s actions reach and impact the situation.
As an example, a situation diagram for a doctor breaching the sterile field during a surgery might be:
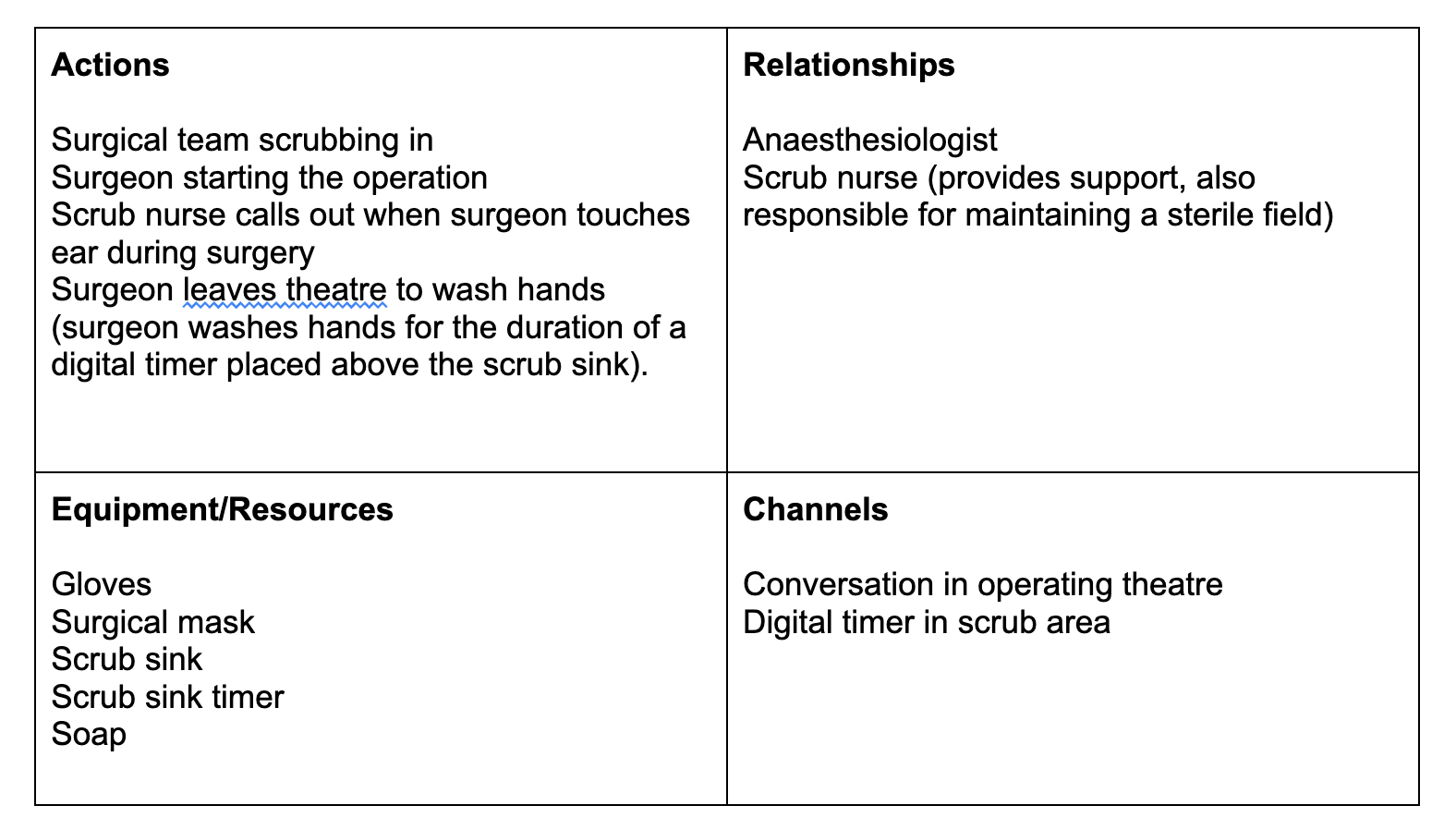
Actions
- Surgical team scrubbing in
- Surgeon starting the operation
- Scrub nurse calls out when surgeon touches ear during surgery
- Surgeon leaves theatre to wash hands (surgeon washes hands for the duration of a digital timer placed above the scrub sink).
Relationships
- Anaesthesiologist
- Scrub nurse (provides support, also responsible for maintaining a sterile field)
Equipment/Resources
- Gloves
- Surgical mask
- Scrub sink
- Scrub sink timer
- Soap
Channels
- Conversation in operating theatre
- Digital timer in scrub area
Let’s pretend that you are a founder who wants to introduce a new device into the operating theatre. The right stance to take when creating this situation diagram is to regard this is ‘business as usual’ — all the elements of the situation are working together to help the various actors cope with their various tasks; you are not yet a factor. You can only insert yourself (and your proposed solution) if there is a gap in the situation: some … thing that actors are currently not coping as well as they could be. The closing of this gap generates a ‘not not’.
The authors write (all bold emphasis mine):
Diagramming situations clarifies how authentic demand arises from within them and how the components of those situations stick together. People in situations cope with them so that the situation remains in place; when gaps open, they act to fill them. So, for example, when a person is a student, they may maintain their grip on being a student by engaging in activities such as attending classes, and they may have a piece of equipment such as a schedule that lets them know the time and place of each class. To maintain a grip on the situation, the student will be nonindifferent to that schedule. If it’s on a piece of paper and they lose it, a gap will have opened up, and they’ll search high and low for it. The authentic demand is for the gap to be closed and the situation maintained, but it’s expressed by the customer as demand for the object, the piece of paper.
For an innovator, an object like a paper schedule, or any other equipment (product or service) is not what’s in demand. The demand is for the gap closing that’s accomplished through that object in a particular situation. It’s convenient but misleading to think of the demand as demand for the object; more precisely, it’s demand via the object for the gap closing. Thinking of the demand as being for the object itself obscures both the size of the market and the risk that the gap may be closed in some other way that wouldn’t be noticed as competitive until it’s too late.
The last sentence is very important, and I recommend rereading it a few times. The implication is this:
- Focusing on the gap forces you to grapple with “how many situations does this gap appear in?” This gives you a sense of the size of the market.
- Focusing on the gap also forces you to ask: “what other ways might people use to close the gap?” Those alternatives may compromise the demand for your proposed product or solution. So for instance, if you want to introduce an AI-driven camera system that will alert the doctor when they breach the sterile field (e.g. when they touch their ear), the obvious counter would be that “I can just ask my scrub nurse to warn me; they are already there, and they are already trained to do this.” The gap isn’t very large; there is no compelling not not.
I will admit that I’m not completely pleased with this language around gaps. I am not certain I could recognise a gap if I found one; the way gaps lead to ‘not nots’ feels slippery to me. For instance, some gaps are created by the introduction of new information into a situation. Pension fund managers were coping just fine before they discovered that their active investors were charging exorbitant fees for index-like returns. You could say that the introduction of this information created the gap.
In other situations, gaps are invisible because existing actors are coping decently, with whatever resources they have available to them. But if you introduce a solution that causes them to cope better, the actor will move to the new solution, and quickly. In other words, exposing customers to a solution that closes a hidden gap in some set of situations should result in a ‘not not’ — as in, you will find that the customer cannot not use your proffered solution, and often cannot imagine operating in that situation in the old way ever again.
Given how slippery gaps are, perhaps you can see why it’s critical to do accurate situational bookkeeping. At Flashpoint, founding teams fill in a small mountain of situation diagrams as they conduct interviews with various stakeholders. In many cases, authentic demand reveals itself in an adjacent situation, not one that involves the initial customer you were targeting. Repeatedly probing these diagrams will help you find unexpected places where you may insert yourself — or your product — into.
But … how should you conduct such interviews?
Documented Primary Interactions (DPI)
At Flashpoint, Furst and Chanoff taught an interviewing process called ‘Documented Primary Interaction’ (or DPI). Flashpoint startups do anywhere between 200 to 400 DPIs over a three to six month period during their search for authentic demand. Founding teams typically start small, and build up to 20 or more DPIs per week.
DPIs must be:
- Documented, so that they can be planned, assessed, and remembered consistently. Documentation includes documenting the design of the DPI as an experiment beforehand and recording what actually happens, preferably by audio or video recording (but if not, through notes written immediately afterward).
- Conducted with people involved in the situation of interest, as distinct from experts or other outsiders opining about a situation they aren’t directly party to. Primaries are people who are actually in situations. Secondaries are people talking about how other people they know or work with operate. Tertiaries are research reports or survey results. DPIs care only about primaries.
- Constructed as an interaction intended to bring out a response that may reveal a non-indifference. This often involves doing or saying something that breaches a norm, in order to test whether or not the person is really indifferent to the change.
In the beginning, you’re just trying to sketch out the situations these people (and businesses) find themselves in. Unlike many interviewing methods, DPIs focus on concrete behaviours and circumstances, not motivations or outcomes. Also unlike other interviewing methods, investigators are expected to pre-register their views before starting a DPI session. These views look like: “What do I expect to find, and what do I expect people to do in a particular situation? What if I change something?” As time passes, and you develop hypotheses for gaps that you might be able to insert yourself into, you begin to probe stakeholders with questions or experiments in order to uncover ‘nonindifference’. So, for instance, you might design an experiment where an actor is tested for price sensitivity. Would they reach for a particular tool if the price was increased? What if it were decreased?
If you’re lucky enough to identify a gap, investigators may start building small prototypes for actors in specific situations. A ‘not not’ is only verified once actors find they cannot not reach for the solution in their specific situations.
All of this is deliberate, time-consuming, and extremely difficult to do. (The authors did name this process ‘Deliberate Innovation’ for a reason). We should be precise about what is so difficult about this process: it isn’t the method that is hard — experienced researchers or go-to-market folks would recognise common interviewing principles in the DPI system. What is difficult is the tendency for founders to lie to themselves. This makes sense: you’ve quit everything to start a company. You want your idea to work.
It can be emotionally difficult to face reality.
In The Heart of Innovation, Furst and Chanoff outline the various cultural and psychological prostheses they had to build into the Flashpoint program in order to enable such truth-seeking behaviours. I’m only going to mention this briefly, because none of these things are going to be easy to inculcate. Amongst other things, Flashpoint startups:
- Practice ‘unconditional positive regard’ — Everyone in the program is expected to show complete support and acceptance of a person no matter what that person says. Self delusion is not a character flaw; it’s simply the way human minds work. Unconditional positive regard in the face of such bias enables …
- The use of Radical Candor — A lot of the work in Deliberate Innovation is noticing and then highlighting one other’s blind spots. When that happens, it’s easy to feel like a fool. The Flashpoint program uses Radical Candor (from Kim Scott’s book of the same name) but make it a point to practice this only in the context of unconditional positive regard. Doing so otherwise is a recipe for disaster.
- Attend to process, and the process cadence — Because innovation work is uncertain, most folks who go through the Flashpoint program feel unmoored. This is understandable: participants want the feeling of explicit progress … but this isn’t possible when you’re doing innovation work. Furst and Chanoff designed their program to focus on the inputs to the process. This is a weekly cadence of, first, planning activities and DPI exercises, then executing those DPIs, then debriefing, presenting and supporting each other as the results come in, and then prepping for the next week’s cycle. This shifts the focus to inputs (which founding teams have control over) instead of outcomes (which teams have no control over).
- Pay attention to indifference — Novice founders tend to look for interest in a potential solution. This is dangerous. Flashpoint teaches its founders to pay attention to indifference. Indifference is everywhere you look, once you learn to see it: most people in most situations don’t care about you or your solution. Becoming sensitive to indifference during the DPI process makes teams more aware of nonindifference when they finally find it.
There are a few more practices in the book, but I’m leaving those out of this essay for the sake of brevity. If you want to put these ideas to practice, I highly recommend buying a copy for yourself, and then reading the chapters in Part 2 very carefully. The upshot is that all of these practices combine to make Deliberate Innovation slightly more palatable. It gives founding teams a better shot of success.
Wrapping Up
The title of this essay reads “why most startups fail”. The implication, of course, is that startups fail when they don’t find authentic demand. Is this true?
There’s a paragraph, fairly early on in The Heart of Innovation, where Furst and Chanoff write (bold emphasis mine):
The biggest problem when creating a new form is being stuck in the world of ghosts. You and your innovation are not being drawn into the world. As smart, energetic, and diligent as you may be, the hard truth is that it’s not up to you to be welcomed; other people have to do it. You have to be let in. You start with a fantasy that people will flock to what you’re doing; it’ll be either love at first sight or at worst a process of educating customers about how your innovation will improve their lives. Like Joe Reger at Springbot (whom we met in chapter 1), we have a hefty to-do list — logos to create, important people to meet, software to debug, pro forma financials to calculate. But then the ghostly reality sets in. People are already coping with their lives: customers are already buying something else, collaborators are collaborating, and investors are investing elsewhere. Your innovation is met with overwhelming indifference. It’s common to swing from one extreme to another, from seeing demand everywhere to seeing it nowhere. That’s a tough swing, psychologically. Plenty of innovators never go even that far; they just keep adding to the to-do list until they run out of time or money or energy, at which point they abandon the innovation and come up with an excuse (usually something about market timing).
At the end of Damballa’s life, a 2016 Security Week article wrote that “‘The company’s technology was too early to the market and required too much customer education to understand the value,’ we were told. Damballa was one of the first companies to use serious machine learning technology to aid in threat detection. But, ‘it’s only been the last three years that the industry and tech buyers have begun to understand the advantages and scale.’”
The authors reject this characterisation. They would say that this was an excuse; the real reason Damballa lost was because it never found authentic demand. And in this, they are in good company.
In his famous product-market fit essay, Marc Andreesen writes:
Lots of startups fail before product/market fit ever happens.
My contention, in fact, is that they fail because they never get to product/market fit.
Carried a step further, I believe that the life of any startup can be divided into two parts: before product/market fit (call this “BPMF”) and after product/market fit (“APMF”) (…)
Whenever you see a successful startup, you see one that has reached product/market fit — and usually along the way screwed up all kinds of other things, from channel model to pipeline development strategy to marketing plan to press relations to compensation policies to the CEO sleeping with the venture capitalist. And the startup is still successful.
Conversely, you see a surprising number of really well-run startups that have all aspects of operations completely buttoned down, HR policies in place, great sales model, thoroughly thought-through marketing plan, great interview processes, outstanding catered food, 30" monitors for all the programmers, top tier VCs on the board — heading straight off a cliff due to not ever finding product/market fit.
Ironically, once a startup is successful, and you ask the founders what made it successful, they will usually cite all kinds of things that had nothing to do with it. People are terrible at understanding causation. But in almost every case, the cause was actually product/market fit.
Because, really, what else could it possibly be?
The biggest form of failure in startups is not finding authentic demand. Because, really ... what else could it possibly be?
Special thanks to Bennett Clement, Vaughn Tan, Crystal Widjaja and Shawn Tan for helping with the structure of this essay.
Originally published , last updated .
This article is part of the Market topic cluster, which belongs to the Business Expertise Triad. Read more from this topic here→