This is Part 2 in a series on learning in ill-structured, novel domains. That said, this post may be read as a standalone.
In our previous members-only post we talked about Cognitive Flexibility Theory (CFT), a theory of adaptive expertise in ill-structured domains. I explained the two central claims of the theory, but I don’t think I did a good job of articulating the core ideas, or why I considered them useful. As a result of several conversations over the past two weeks, I think I’ve figured out a way to explain CFT more compellingly; consider this piece a rewrite of last week but with the actionable recommendations added at the end.
Cognitive Flexibility Theory is a 30 year old learning theory that ends up someplace weird: it tells us that a specific type of note taking will help us learn better from history. In the process, it explicates how expertise works in ill-structured domains, it pokes holes at the primacy of first principles thinking, and it explains how to properly learn from experience.
In order to get to all of this, we’ll need to start at the beginning.
If you’re a long-time reader of Commonplace, you might already recognise the name. CFT is one-half of the two theories that underpin the work in Accelerated Expertise , the best book on accelerated training programs we have today, originally prepared for the US Department of Defence and published in 2016. (If you haven’t read my summary, you might want to give it a go; AE was one of the more remarkable books I read last year. The other theory from AE, Cognitive Transformation Theory, is covered elsewhere in this blog).
CFT deals with a very specific aspect of expertise. It asks: “how do experts deal with novelty?” The proper term for this subfield of expertise research is known as ‘adaptive expertise’, and it was originally established by a landmark 1988 paper called Two Courses of Expertise, by Giyoo Hatano and Kayoko Inagaki. The observation that Hatano and Inagaki made goes something like: “Sure, sure, we have plenty of studies of ‘classical expertise’ now, where people play chess or make the same sushi roll hundreds of times to perfection. But what about the cases where a grandmaster invents a new opening, or a sushi chef comes up with a completely new menu? What can we say about that? How do we train students to do that?”
If you think about it for a moment, adaptive expertise colours a lot of what we expect to see in our careers. We are often asked to improvise. We are often presented with novel situations to solve, due to the specific constraints of our business, our industry, or our customers. As an operator, no situation that you face in the real world will perfectly match the frameworks articulated in business books. As Hatano and Inagaki puts it: how do you train to get better at that?
As far as I can tell, CFT is one theory that has gone very, very far in answering this question. The primary caveat with CFT is that it is built off work done in accelerating advanced medical education — think: junior doctors, faced with patients in the hospital system, not first-year medical students in the lecture hall. Everything I’m about to describe should be read with this caveat in mind.
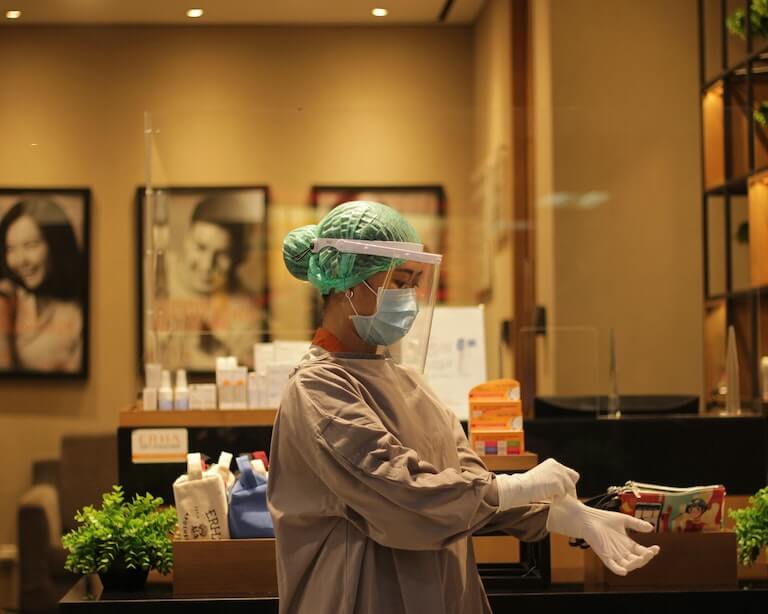
The Four Big Ideas of Cognitive Flexibility Theory
CFT has two primary claims, but there are two big ideas that we must examine first, before we get into the theory proper. We’ll look at them in order.
Idea One: CFT is Concerned With Ill-Structured Domains
The key domain feature that CFT grapples with is something that the authors call an ill-structured domain. An ill-structured domain is a domain where there are concepts, but the way those concepts are instantiated in the real world are hugely variable, and messy as hell. As a result, most cases that practitioners deal with in an ill-structured domain will be novel.
For instance, think about heart attacks. A heart attack is a concept. It is a thing you can study in medical textbooks. But actual diagnosis of heart attacks can be hugely variable — it depends on the patient’s race, age, gender, case history, potential complications, and so on; some heart attacks present as indigestion initially, others may last days.
As an example of what heart attack recognition looks like, here’s a story from Sources of Power:
A paramedic described a family gathering where she saw her father-in-law for the first time in many months.
“I don’t like the way you look,” she said.
“Well you don’t look so great yourself,” was his answer.
“No, I really don’t like the way you look,” she continued, “We’re going to the hospital.”
He grudgingly agreed to go the next day, but she insisted they go right then. An examination showed a blockage to a major artery. By the next day, he was having surgery to clear the blockage.
And notice the difference between concept and concept instantiation:
Many of us view the heart like a balloon. A person is walking along just fine and then, ping, something snags the balloon and the person goes down with a heart attack. This metaphor is not accurate. The heart is a pump, with thick, muscular walls. It does not burst, like a balloon. Instead, it clogs up, like a pump. Sometimes it clogs up quickly, as when a clot lodges somewhere (here’s where the balloon metaphor may come in). When it clogs up slowly, during congestive heart failure, there are (other) signs. Areas of the body that are less important get less blood. By knowing what they are and by being alert to patterns in several of these areas, you can detect a problem in advance. The skin gets less blood and turns greyish. That is one of the best signs. The wrists and ankles show swelling. The mouth can look greenish. Our interviews with physicians, paramedics, and others turned up these indicators and several others.
This is an example from medicine, though. Let’s talk about business, another ill-structured domain. Consider ‘scale economies’, a competitive advantage we’ve discussed before. A novice might read ‘scale economies’ and think “ahh, this occurs when the unit cost per customer goes down with scale.” They might even fixate on a manufacturing example.
But consider the following two cases:
Case One: Texas Instruments — In the late 60s, then-Texas Instruments VP Morris Chang noticed that there was a learning period in the beginning of every semiconductor manufacturing run, where they would struggle to get yields up for each new process. The conventional wisdom at the time was to charge a high price for the chips from the get-go, since there was so much capex involved in manufacturing a new process. Chang thought this was silly. He hired a bunch of BCG consultants and told them to look into pricing the chips for volume. Eventually they came up with something called ‘learning curve pricing’ — TI would initially price the chips cheaply, capturing a ton of market share and driving volumes up to max capacity, which then allowed Chang and TI’s other engineering staff to rapidly climb up the learning curve in order to increase yields (and therefore margins). TI would then make the chips for as long possible to recoup the initial fixed costs, helped by its dominant market share. Chang said, of the time: “We would automatically reduce, and then continually automatically reduce the price every quarter even when the market did not demand it. This was a very successful effort, even though it was somewhat controversial. A lot of people thought we were being foolish. Why would you reduce the price when you didn’t have to? But we did it because we believed in it, and indeed our market share just kept expanding. That, combined with other strategies, made the TI integrated circuits business the biggest IC business in the world, and also the most profitable.” (Source)
Case Two: Netflix — Netflix began ramping up its debt load in 2011, going from slightly under $1 billion dollars in 2014 to $16 billion dollars of debt in 2020 — mostly in the form of junk bonds. This was a remarkably large (and arguably risky) bet, but Netflix needed the cash in order to transform itself from a streaming provider to a content producer. In 7 Powers, Hamilton Helmer wrote about Netflix’s strategic pivot like so: “On the face of it, Netflix’s moves looked risky, overly ambitious. Creating originals and thus tying up all the rights to that content was more expensive. Further, Netflix had previously been down the road of original content with its Red Envelope Entertainment, and the results weren’t pretty. So too did it seem now that such forward integration might prove “a bridge too far.” But these bold, counter-intuitive moves proved game-changing. Exclusive rights and originals made content, a major component of Netflix’s cost structure, a fixed-cost item. Any potential streamer would now have to ante up the same number of dollars, regardless of how many subscribers they had. If, say, Netflix paid $100M for House of Cards and their streaming business had 30M customers, then the cost per customer was three dollars and change. In this scenario, a competitor with only one million subscribers would have to ante up $100 per subscriber. This was a radical change in industry economics, and it put to rest the spectre of a value-destroying commodity rat race.”
Notice how both cases are instances of ‘scale economies’, but each case is expressed very differently. One involves a manufacturing learning curve, which is itself different from the textbook example of lower unit costs at scale; the other example takes advantage of cheap debt, advantaged access to capital markets, and the dynamics of the streaming industry circa 2011. In other words, Netflix could turn its size into an advantage because of its pole position in the streaming wars; it’s difficult to talk about scale advantages in its case without also talking about the conditions that allowed it to execute this strategy in the first place.
To wrap up, the formal definition for ‘ill-structured’ is “concept instantiation is highly variable for cases of the same nominal type”. If you think about your domain for a bit, you’d probably realise that some parts are ill-structured, while others are not — for instance, in software, computer programming is well-structured but software project planning, software design, timeline estimation and security event mitigation are rather ill-structured. Hell, if you think about any career or any industry, you’d probably realise that important aspects of it are ill-structured. That leads us into our second idea …
Idea Two: In Ill-Structured Domains, Cases Are As If Not More Important Than Concepts
CFT asserts that due to this nature of ill-structured domains, cases are as if not more important than concepts. This second idea is a more subtle one, so we’re going to spend a bit of the time examining the implications.
I think many of us have been exposed to a particular style of teaching in school, where we are taught a concept, and then the examples that illustrate that concept are treated as disposable. My go-to example for this is how we are taught quadratic equations — we are shown one or two examples and then we are expected to memorise the general approach for solving such equations. As a result of this instruction, many of us internalise that concepts are important and examples are not.
This is even more pervasive for those of us who have STEM backgrounds — where we are taught that principles are everything. This is why, for instance, you’ll see engineers evaluating quality of thinking with a dismissive “you’re not thinking from first principles” — the assumption being, of course, that reductive reasoning (that is, breaking things down to root issues) and principled logic are paramount in any real world analysis.
Incidentally, this is also the way that I was trained to think and argue — and perhaps the way that many management consultant-types are trained to think and argue: you make an assertion, back it up with some reasoning, and then give one or two illustrative examples, keeping in mind that you may drop the examples if you run out of time.
But CFT challenges this view. It points out that if concept instantiations are highly variable in an ill-structured domain, then reasoning from first principles is very difficult.
How do we know this? I mentioned earlier that CFT comes from the study of accelerating medical expertise. Multiple precursor studies that led to CFT show us that:
- Journeymen doctors are unable to identify cases when they are taught the concept alone.
- They are not able to go from symptom presentation back up to the concept and mechanism of disease.
- There is this tendency for novices to cling to the generalised lessons from one case, and then struggle when presented with a concept instantiation that is very different from the prototypical case they hold in their heads.
- And indeed, experts in ill-structured domains reason by comparison to previous cases, not by reference to first principles. (Source: see citations in the original CFT paper)
If you don't believe that last point, consider the two examples of scale economies earlier. What first principles might you extract from each example? Is it that ‘experience curves benefit from high volume so you should price for maximum production capacity’? But then what if everyone in your industry already understands this and has priced accordingly — as was the case when Morris Chang went off to start TSMC? Or perhaps ‘issue junk bonds to turn your variable costs into fixed costs when you are in the pole position in your industry and your competitors haven’t realised that you can do this’? These ‘takeaways’ sound almost contrived — and are in fact likely overfitted to the idiosyncratic details of each case.
The truth is that you can’t easily reduce cases in ill-structured domains into generalisable principles. You often have to treat the case as its whole thing.
Of all the ideas in CFT, this is the one I struggle with the most.
For instance, I continue to believe that first principles thinking is necessary for good problem-solution analysis. In business, you’ll often find yourself faced with an ill-structured problem to solve: your churn rate is higher than expected; your sales productivity stinks; you can’t seem to hold on to people for longer than a year. In these situations, it’s tempting to do some lazy pattern-matching and then dive straight into plausible solutions. I’ve nearly always regretted it whenever I’ve done this. I have found that it is more useful to break things down from a ‘plausible root cause’ perspective, and then rank solutions in the order of how much information the solution might generate towards determining which set of plausible root causes might be in play. In other words, I think from first principles. So obviously I continue to believe that first principles thinking is good, and has its place in the operator’s tool belt.
But I have to admit that CFT’s focus on the primacy of cases resolves a number of long-standing questions for me.
Consider this, example one: I read a lot of business biographies because I want to become a better businessperson. What lessons should I take away from the story of Facebook, or Intel, or TSMC? In other words: why should we study history, given that history doesn’t repeat itself, and in an ill-structured domain like business, all experiences I have will be novel?
The answers commonly given in response are things like “so you may have more patterns to compare against”, and “to build context.” But this just begs a series of follow-up questions: “why is context useful?” and “how can you expect to use pattern-matching when faced with novelty?”
CFT gives us a more coherent answer to these questions, which we’ll get to in a bit.
Here’s example two: I’ve long puzzled over Charlie Munger’s thinking style. Munger is Warren Buffett’s business partner, and a legendary investor in his own right. He has this thing where he says that you must have a ‘latticework of mental models’ in your head if you want to be a great stock picker. If you are a long-term reader of Commonplace, you’re probably familiar with my critique of the mental models obsession that seems to have overtaken self-help land. Plenty of writers have adopted Munger’s approach to an extreme: they go on and on about context-free mental models without ever asking: how does Munger actually use those models in practice?
And so therein lies the puzzle: in the years since I first investigated the whole mental models shebang, I’ve read a lot by Munger and about Munger — and as far as I can tell … Munger spends a lot of time reasoning by analogy.
I’ve written about this before, in my summary of Range:
“We want to win a position in the legal research market with analytics and, as lawyers turn to us for unique insights and easier user interfaces, we can expand our tools and content until we’re a complete alternative to the incumbents. Our technology scales efficiently, so we can also offer lower prices.”
Charlie spotted another pattern. “This reminds me of the ‘Cola Wars’ between Pepsi and Coke. Up until the Great Depression, Pepsi and Coke were priced the same and Coke was dominating the market. But then Pepsi cut their price per ounce by half and their sales took off, with profits doubling too. Price can be a powerful competitive tool when you have a good substitute product.”
Eventually, Charlie asked us for more detail about our funding plans. We told him about the round we were assembling and some of the other investors involved.
“I bet” he said, “that if I invested I could be a Judas goat for you.”
He noticed our confused looks.“You’re not familiar with a Judas goat?”
We shook our heads.
“A Judas goat is the goat that they lead from one pen to another, or the slaughterhouse, that all the other animals follow. I bet if I invested, you’d have a lot of other investors that would sign up too.” (source)
I always found this confusing. Isn’t first principles thinking The Best Way to Think? And so why is it that this remarkably intelligent, remarkably wise man is so keen on reasoning from analogy?
The answer to this question brings us to the final two ideas of the theory …
Ideas Three and Four: The Two Claims of CFT
CFT makes two central claims. How do experts deal with novelty in ill-structured domains? In other words, what lies at the heart of adaptive expertise? CFT tells us that experts do two things:
- They construct a temporary schema on the fly, by combining fragments of previous cases.
- They have something the authors call an ‘adaptive worldview’: meaning that they do not think there is one root cause or one framework or one model as explanation for a particular event that they observe in their domain.
This second point is a little subtle, so we’ll need to expand on it a bit.
When you or I (that is, non-doctors) think of a heart attack, we probably imagine a prototype of a heart attack in our heads. That is, we imagine what we think is an ‘ideal’ heart attack, where a person falls over and clutches his or her chest. Expert doctors do not do this. They do not reduce a concept like heart attack to just one prototype. They instead have a collection of prototypes in their heads, that they can assemble fragments from.
What an adaptive worldview means is that whenever you learn a new concept in an ill-structured domain, you know not to oversimplify — that is, to represent it as a single principle or concept. You do not try to reduce. You instead know to search for new, different cases in order to collect a cluster of prototypes in your head, and let that cluster inform your understanding of the concept. If you encounter a new case, you update the concept, because the concept is only useful when you know how it is instantiated in reality.
(Notice that the claim is that experts think like this — they may well articulate a simple principle when you ask them, but that is not how the concept is represented in their heads.)
This is a very subtle distinction, so I’ll let the authors of CFT describe the adaptive worldview:
(Experts in ill-structured domains) … pay attention to cases in the variegated richness while de-emphasising the primacy of concepts (which serve a needed subsidiary function to cases in ill-structured domains); use multiple rather than single conceptual relations (as in schemas, prototypes, analogies, perspectives, etc); treat cases as wholes with emergent properties so they are greater than the sum of their parts; increase the attunement to difference and decrease the bias toward seeing similarity; expect unpredictability, irregularity, contingency, indeterminateness; expect to return to earlier cases in new contexts to bring out facets that were hidden in the earlier context — nonlinear revisiting is not repeating; embrace flexibility and openness of knowledge representation over rigidity; stress context dependency over context independence; avoid rigidity in understanding, remaining open instead, with an appreciation for the sometimes limitless range of uses of knowledge in new combinations, for new purposes, in new situations; rely on situation-adaptive assembly of prior knowledge and experience rather than retrieval of intact knowledge structures and procedures from long-term memory … (taken from page 962, The Oxford Handbook of Expertise)
Using Cognitive Flexibility Theory
Now that we have the four big ideas of CFT, we can turn to the actionable question: “how do we use this?” The answer is that we take the two claims of the theory and invert them to get the pedagogical recommendations:
- You want to expose the student to as many cases for each concept as is feasibly possible, so they have a large collection of fragments to assemble from.
- You want to inculcate the adaptive worldview.
How do the researchers recommend doing this? One problem with studying cases is that humans aren’t great at remembering all the ‘variegated detail’ of each case — and each case often has a large number of concepts interwoven into the history. So the researchers recommend using a hypertextual system — that is, a system where you can link to other notes, or link to tags that in turn link to other notes. You get the student to store each case and ask them to highlight concepts. Concepts are backlinked. They go to other cases.
There are many variations to this approach. Many CFT learning systems come pre-loaded with cases, marked up by expert doctors or practitioners. Students are given an initial case that is particularly rich with highlighted concepts and features (the researchers call these cases ‘crossroad cases’, about which, more in a bit). They are then asked to explore the system by jumping from case to concept to other case.
The hypertext nature of the system explains how students get to compose fragments from many different cases. But how do you inculcate the ‘adaptive worldview’? In their chapter in the Oxford Handbook of Expertise, the researchers say that they’ve found four ways:
- You give the student an overview of the CFT mindset — basically, something like this piece that you’re reading, before you let them explore the system. (Jonassen, Ambruso, and Olesen, 1992)
- You have the system display a mantra. For instance, CFT instruction frequently invokes mantras like “it’s not that simple”, or “it depends”, followed by presentation of a follow-up case that is very different from the first.
- You design a four-stage model for worldview change into the learning system. The four stages go like this — first: demonstrate to a learner that they have a reductive worldview by creating a situation that makes it salient (say, the heart attack diagnosis example, above, where you want to get the student to fail at some task). Second: show how that worldview is maladaptive (show them that the metaphor of the heart as a balloon is flawed). Third: introduce the adaptive worldview and its properties. Fourth: demonstrate the latter’s operation and then provide an activity for mastering it. (Spiro et al, 2007)
- Finally, exploring the CFT system itself inculcates the adaptive worldview. As a student explores the hypermedia system, they realise that there is huge variability in the way a single concept is instantiated in the real world.
How do you construct a CFT hypertext system for yourself?
The CFT summary in The Oxford Handbook of Expertise has a nice section on how one might construct a CFT learning system for yourself. I’m going to give you an adapted version which I’m experimenting with for marking up business cases, which does not assume that you can code. But keep in mind that I’m new to this; give me a few months before I write up some notes from practice.
Step One: Pick a note taking app with backlinking capabilities. Backlinking is a feature where you can select a phrase, perhaps ‘scale economies’ or ‘heart attack’, and turn that phrase into a link — something that looks like [[scale economies]] or [[heart attack]]. Clicking the backlinked phrase brings you to an interface that shows you all the other notes that have been linked with ‘scale economies’ or ‘heart attack’, which means that you can implement the ‘case’ -> ‘concept’ -> ‘case’ reading pattern described above. Note that it doesn’t matter which note taking app you use; what is important here is the particular style of cognition that is promoted by the CFT learning system. Popular apps include Obsidian, Logseq, Roam and Craft — just pick one and go.
Step Two: Start copying cases into your note taking app, perhaps from articles, PDFs, books or blog posts. Mark up particular passages with concepts or case features that you observe. As you do so, split up the passages into smaller segments, that will hopefully show up in your backlink interface.
The purpose of the segmentation is so that you may just grok a part of a full case when you are doing revision. You shouldn’t need to reread the full case if you are doing a conceptual search on your notes — so fragments are important.
This highlighting and segmenting bit is a bit tricky, because proper CFT learning systems ask expert practitioners to mark up cases for students. Presumably, as a novice learner, you’re going to miss certain concept instantiations or cues that an expert would catch. But I think this is ok — this is the best that one can do when you’re creating a CFT system for yourself.
Do not worry so much about breaking up the cases the ‘right way’ — the researchers stress that there is no one ‘right way’ to turn cases into fragments. (In fact, they say “even dividing at convenient seams work roughly as well” — it’s really up to you!) What you shouldn’t do, though, is to attempt to organise cases into clearly defined, homogenous stages — which people tend to do when there is a temporal element to a domain, for instance the presentation of symptoms in a patient’s case history over time. This is because cases in ill-structured domains can differ even if most cases follow the same underlying progression or structure!
How do you find cases to add? The researchers recommend starting with ‘crossroad cases’, that is — cases ‘rich with conceptual features that are crucial to the domain’ and together ‘could even be considered emblematic of the domain’. The researchers recommend a starting set of 10-20 such cases — in fact, all CFT learning systems come preloaded with a collection of 10-20 crossroad cases.
This in turn means that you must look for initial cases that are as different as possible from the ones you currently have. And it means that you should continue looking out for such rich cases until you reach diminishing returns, where your core collection of crossroad cases may be said to be representative of the problem domain. So for instance, if you’re creating a learning system for heart attacks, you’ll want to have 10-20 cases that represent the most important variations from real world diagnostic practice.
Step Three: So far we’ve talked about what goes into the system. But how do you use the system to learn?
As far as I can tell, CFT learning systems work through two modes, both of which are expressions of ‘combinatorial idea play’:
- You give the student access to a CFT learning system, and then present them with a series of tasks to do (I imagine this as a doctor being presented with a series of increasingly difficult cases — “Male, 63, found at the bottom of a flight of stairs with multiple contusions …”, and evaluated using diagnostic questions). The CFT learning system is their reference; their job is to do a concept search across the entire case library until they find the relevant fragments and give their best guess answer.
- You get the student to do multiple case contrasts, in order to build complex understanding more rapidly. How you encourage this: you give them a series of questions like “how is Case A like Case B but not like Case C” and “find surprising differences between cases that appear similar, and find surprising similarities between cases that appear different on the surface” and so on — thus reflecting the nuances of expert understanding. A variant of this is to see how what is most important about a previous case changes in the context of other new cases (one mantra of CFT is that “revisiting is not repeating”).
Both methods force students to practice ‘schema assembly of fragments’. And they do one more thing: they get students to overlearn the crossroad cases!
Let’s talk about crossroad cases now, why they are important, and why they are named that way. As a student engages with a CFT learning system, they will encounter the richest 10-20 cases again and again. In other words, as they jump from case to case, they will likely criss-cross the same richest cases during their system traversal (hence the name ‘crossroads’ cases!) Recall that such cases are chosen because they are ‘emblematic’ of the domain. This produces three things:
- The crossroad cases will quickly become the most analysed — meaning that the student will, as part of their tasks, unpack a crossroad case in as many ways as possible, producing deep learning for that case and internalising that cases are complex and a case is not just a case of one thing, but has many possible ‘titles’ — that is, cases in ill-structured domains are cases of many things.
- Second, as crossroad cases are reused in new contexts, they quickly become ‘overlearned’. What this means is that a student will become so familiar with the crossroad case that even reading a small fragment of it will evoke the rest of the case, cognitively bringing the entire case history ‘along for the ride’. The researchers call the small distinctive fragments ‘epitomés’, and they call this stage ‘epitomé mode’. Once epitomé mode is reached, case combination and case contrasts can be done at the speed of thought. Effectively, this also means that the student has a set of cases stored in their memories available for invocation and assembly — just like a more experienced practitioner would!
- Finally, crossroad cases are powerful because they promote connection building between many concept instantiations. This is mostly done through the ‘case contrast’ method we covered above — each crossroad case is treated as a hub, and the student is asked to find as many connected cases on as many conceptual dimensions as possible (the spokes). Then a new crossroad case is selected as a hub, and the student repeats the process.
This is all well and good, I hear you say. But what activities can you do when you’re constructing a case library for yourself? This is a good question, and I’m not entirely sure yet — give me a few months to test this in practice for myself.
The Most Impressive CFT System I’ve Seen
One last note, before we wrap up. I think the most impressive system I’ve found while reading up on CFT is the one written about in this paper, titled Reflections on a Post-Gutenberg Epistemology for Video Use in Ill-Structured Domains.
The paper describes a video system that implements a CFT-type pedagogy. The authors describe this system:
So, for example, in one CFT-based video system (Palincsar, Spiro, Kucan, Magnusson, Collins, Hapgood, Ramchandran, & DeFrance, in press) to teach reading comprehension strategies, the thematic concept of scaffolding would be taught by first having the learners look at a large number of examples of scaffolding in instruction. They then come to see that scaffolding is a very complex concept for which they can not prepackage a definition that would adequately guide use. And they also see demonstrations of the rich variety of contextual features that affect how the concept is applied.
One of our interface features, the “Weave” mode (which allows four video clips to be compared in simultaneously appearing quadrants – this feature can be seen in EASE History, at the URL provided earlier), permits a certain kind of exercise that is useful for helping people to understand the ill-structured character of such conceptual families. We ask people to use the Weave interface to set up four clips that belong to the same conceptual category and then to identify surprising similarities (clips that don’t appear similar on the surface but on closer inspection can be seen to be instances of the same concept) and surprising differences (aspects of clips that seem similar on the surface but that are different in interesting ways when viewed more closely). Learners are quickly dissuaded from reductive notions of meaning and concept-use; and a richer sense of meaning for the particular concepts is provided.
The researchers talk about reading comprehension strategies, but my mind immediately went to game tape. I’ve often found it irritating to review Judo video when attempting to learn a new technique — I’ve had to manually keep track of technique variations in a note taking app, with links to specific Youtube videos or timestamps. And nearly everything that Spiro et al describe in their research is applicable to Judo as well — throws may seem similar, but setups, grips, entries, and execution are hugely variable for some throws. As an example, the Judo throw Uchimata has at least six entries and five different grips, to be used in different fight situations, despite being the exact same throw at a conceptual level.
Now take this interface, and this learning theory, and apply it to Dota, say, or football, or even computer programming streams. Let’s say that we can mark up video segments with conceptual metadata, which means that we can jump from video to concept to player, to style, and so on. Let’s say that we may slow down replay to compare between videos in a four-quadrant interface. What learning experiences might be made possible with this approach?
Wrapping Up
I’ve presented a 30-year old theory that explains how to learn better in ill-structured domains. We ended up at a surprising place: a note-taking learning system to accelerate expertise. In the process, we took a look at expert performance under novelty, we poked holes at first principles thinking, and we examined features of expertise in ill-structured domains — features that make it rather difficult to reach mastery.
I must admit something at this stage. I have long resisted the notion of better note-taking as a method to do better thinking, much less as a way to rapidise the acquisition of expertise. This is the first time I’ve seen a system that a) has a track record of implementation, b) has a coherent explanation of the underlying cognitive science of learning, and c) is able to explain how it might achieve results in a messy domain. Colour me impressed.
How much more is there to find out about CFT? What may we learn from the past 30 years of system implementations? What system failures and blind spots are there?
I don’t know, but I’m going to find out.
This is Part 3 of the Learning in Ill-Structured Domains Series. Read the next part here.
Sources
- The single best resource for CFT is probably the summary chapter in The Oxford Handbook of Expertise. This isn’t available online, though I’ve included a scanned copy for members at the bottom of the previous (members-only) post.
- Cognitive Flexibility Theory: Advanced Knowledge Acquisition in Ill-Structured Domains by Spiro, Coulson and Feltovich is the original 1988 paper. Originally published in Proceedings of the Tenth Annual Conference of the Cognitive Science Society.
- Reflections on a Post-Gutenberg Epistemology for Video Use in Ill-Structured Domains is the most impressive system implementation I’ve found so far.
- Jonassen, D. H., Ambruso, D. R., & Olesen, J. (1992). Designing a hypertext on transfusion medicine using cognitive flexibility theory.
Originally published , last updated .
This article is part of the Expertise Acceleration topic cluster. Read more from this topic here→