This is Part 4 in the Learning in Ill-Structured Domains Series. Read the previous part here.
Two weeks ago we discussed Cognitive Flexibility Theory (CFT), a theory of adaptive expertise in ill-structured domains. The question the theory grapples with goes something like “how do experts deal with novelty in their domains — that is, things that they’ve never seen before?” We talked about how the answers to that question gives us pointers to better note taking, explains how learning from history can be instrumentally useful, and explicates how experts in ill-structured domains reason by analogy (as opposed to first principles). A week later we discussed several second order implications of the theory — that is, ideas that are bound to come up if you want to put the theory to practice.
This week, we’ll take a look at some of the caveats.
Concepts ARE More Important in Well-Structured Domains
In my original piece about CFT I made a big deal about how learning from cases are as if not more important than learning from concepts in ill-structured domains. I said that this was notable because it went against the style of teaching we’re so used to in school, where concepts are often presented as the more valuable thing; examples are usually treated as disposable.
There’s an important caveat to this idea, though — which is telegraphed by the clause ‘... in ill-structured domains’, above. In well-structured domains, concepts are still more important than cases, and reasoning from first principles is usually more effective than reasoning from analogy. Which is to say that CFT makes all sorts of interesting, unorthodox recommendations for learning in ill-structured real-world domains, but don’t you go applying its ideas to math, or computer programming or, hell, the study of French.
The formal definition for ’ill-structured domain’ is “concept instantiation is highly variable for cases of the same nominal type” — in other words, your domain may have concepts like ‘competitive advantage’ or ‘returns to scale’ or ‘heart attack’, but the way these concepts show up is hugely dependent on the context.
But not every domain is ill-structured. Math and French and computer programming are all remarkably well-structured domains: the way you solve a quadratic equation is the same regardless of whether you’re taking an exam today, or tomorrow, or the year after; a Python for-loop works the same way in every Python program you write. Similarly, while the rules of French are rich and somewhat varied — as it is with all natural languages — they do not change with every sentence that you utter.
(Yes, those of you who aware of language pedagogy might object at this point. As we’ll soon see, learning second languages is a bit of an in-between thing — somewhere between well-structured and ill-structured, with a bit of both elements represented in the learning theories over the past four decades. But I’ve chosen the example of language learning for a reason and we’ll get to that in a bit.)
Contrast these examples with software engineering. Over time, an experienced software engineer might acquire several heuristics so that they may forecast feature timelines more accurately, but every feature is its own unique thing. In order to forecast for a specific task, the engineer would have to grapple with many different factors: the state of the codebase (some better than others), the complexity of the feature (some harder than others), the ambiguity of the problem domain (do we know what we really need to solve?) and the information environment that the team operates in (would the engineers working on the feature have the knowledge they need to complete the feature?)
As a result of all this variation, CFT reminds us that you often can’t generalise from case studies. It also tells us that attempting to go the other way — that is, attempting to apply ‘universal’ principles that are actually context-dependent — often results in failure. And so what CFT suggests that you must do is surprising if you are well-adapted to the traditional education system: it tells you that experts in ill-structured domains reason by analogy to prior cases; it argues that you should study cases in all its variegated glory without abstraction, and that you should hold a cluster of cases in your head so that you may reason by comparison to fragments of the cases you’ve already seen.
You should notice, however, that all of CFT’s recommendations are somewhat inefficient. In fact, I would go so far as to argue that if you’re in a well-structured domain, the recommendations espoused by CFT should be regarded as grossly inefficient — inefficient to the point where you should shy away from its ideas. This is intuitive, I think. If you have concepts, and your domain expresses those concepts clearly — then by God, go learn the concepts. You’ll save yourself a truckload of time if you can reduce a large selection of cases down to a simple principle or two.
I say this mostly because it is tempting to read CFT and say “cases are more important than abstract principles!” and champion the power of analogical thinking over first principles thinking. But it really depends on the domain. Much has been written about Elon Musk’s love for first principles — to the point where self-help sites proclaim first principle thinking to be the secret to Musk’s effectiveness and success. But the majority of concrete examples I’ve seen where Musk breaks something down to first principles and succeeds has often been in the domain of some more well-structured thing — hardcore rocket engineering, say, or upending decades of industry dogma by calculating the ‘actual’ bill of materials for the manufacture of a starship. It’s no accident that engineer-types often espouse the primacy of first principles thinking: when you’re problem solving in engineering, it’s usually first principles reasoning that gets you the furthest.
Ok, ok, I hear you say. Engineering is all well and good. Now what about language?
I referenced learning French earlier for a good reason. There are, broadly speaking, two primary approaches to language learning. The first approach is to learn the rules of the language — the grammar, along with some vocabulary, blended together through instruction, drilling and practice. This is the kind of teaching you’re most likely to get if you attend a language course today. The second approach is to learn as a toddler would: that is, exposure to a large amount of samples, a focus on communication over correctness, and then, through sheer volume, the learner eventually develops an internalised sense of the language’s grammatical rules. I’m skipping over a long history of pedagogical development here by describing just two primary approaches — my point is that language learners around the world learn by some combination of the above two methods, and that there’s a certain amount of similarity to our discussion of cases (language samples) vs concepts (grammatical rules) that we first saw in CFT.
What to make of this? At this point I think it is useful to think of structuredness as a spectrum. If I were to plot an imaginary line in my head, computer programming and math would end up on the well-structured end of the spectrum; business would end up on the ill-structured end. Language learning lies somewhere in the middle. This is a long-winded way of saying that languages mostly follow some set of grammatical rules, but also display some amount of variability in usage (if we were on Twitter and I wrote “it me” while quote-tweeting a picture of a satisfied cat, you would understand that I am making a lolcat joke, and that I’m not necessarily terrible at grammar).
I think the common practitioner argument for optimal language learning is to do a bit of both — i.e. if you want to get to a high level of proficiency in French, the pragmatic approach is to do a combination of both concept learning as well as naturalistic ‘talk to lots of French people and piss them off’ learning. The generalisable lesson, if there is one: if there are concepts that instantiate themselves consistently in your domain, learn them. CFT simply explains what experts do when that isn’t possible. Amongst other things, it explains why learning strategies that work so well in the classroom (and in test-taking environments) often don’t produce the same positive outcomes in the real world. Ill-structured domains simply call for a different approach.
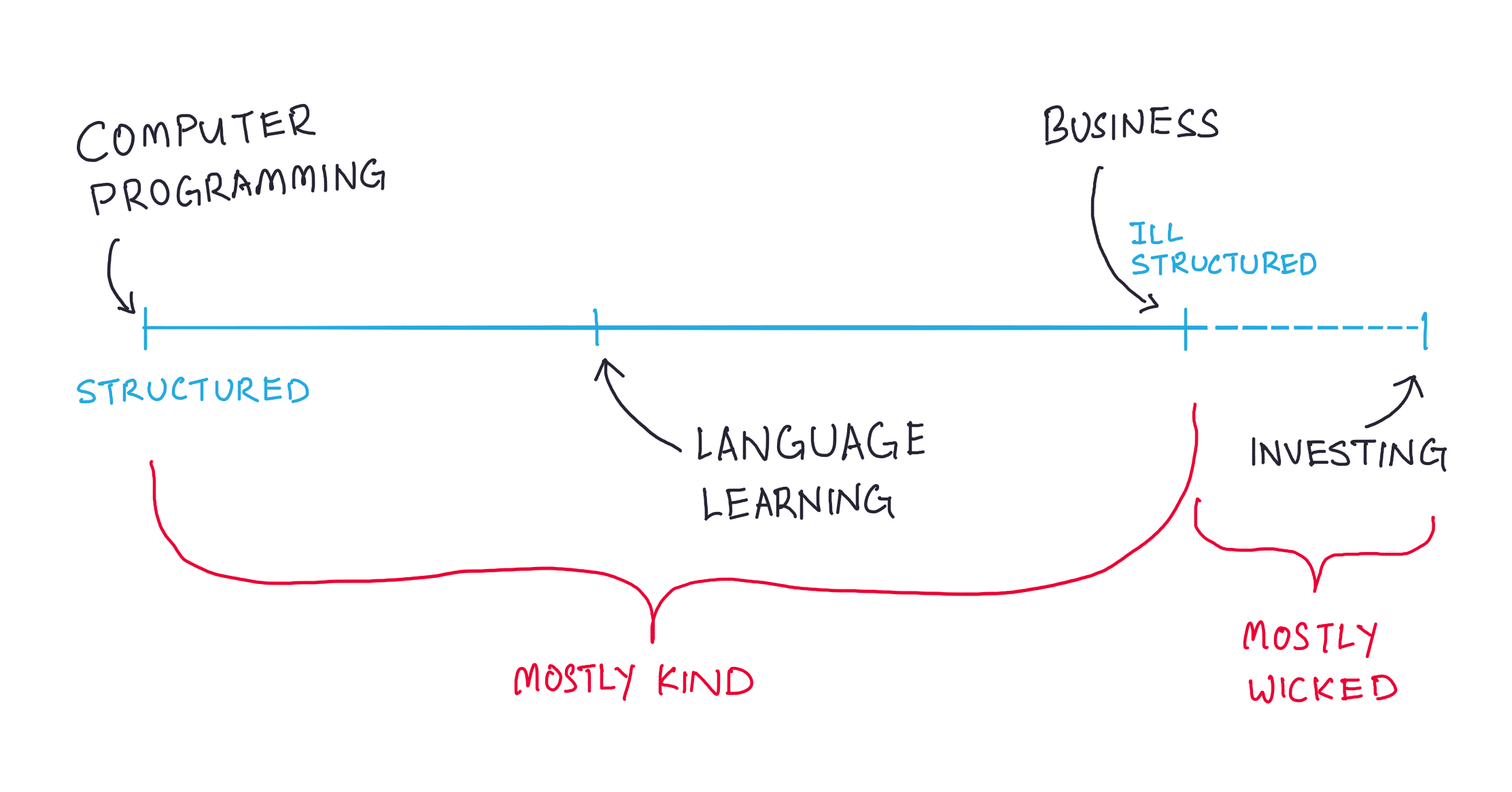
One final note: you might notice that I’ve put investing at the far end of the spectrum, beyond the example of business. Oh, you might say. Why is investing there? I think that’s worthy of a separate discussion on its own.
Ill-Structured Domains Aren’t Necessarily Wicked
In his 2001 book Educating Intuition, psychologist Robin Hogarth introduced the notion of ‘kind’ vs ‘wicked’ learning environments — that is, the idea that certain environments could lead to effective expert intuition, while other, more ‘wicked’ environments lead to erroneous intuitions and therefore hubris. The ‘kind’ vs ‘wicked’ dichotomy was an idea that was reused by Gary Klein and Daniel Kahneman in their landmark Conditions for Intuitive Expertise paper, and it was again popularised in David Epstein’s popsci book Range.
I’d never read Hogarth’s book — at least not before prepping for this piece. If I only went by Epstein’s writing, or by Kahneman and Klein’s arguments in their 2009 paper, I’d have thought that Hogarth was making an argument about ‘kind’ vs ‘wicked’ properties that were innate to a particular domain. For instance, Kahneman and Klein argued the following in their paper:
The recognition model implies two conditions that must be satisfied for an intuitive judgment (recognition) to be genuinely skilled: First, the environment must provide adequately valid cues to the nature of the situation. Second, people must have an opportunity to learn the relevant cues.
They then threw shade at stock picking:
Validity, as we use the term, describes the causal and statistical structure of the relevant environment. For example, it is very likely that there are early indications that a building is about to collapse in a fire or that an infant will soon show obvious symptoms of infection. On the other hand, it is unlikely that there is publicly available information that could be used to predict how well a particular stock will do—if such valid information existed, the price of the stock would already reflect it. Thus, we have more reason to trust the intuition of an experienced fireground commander about the stability of a building, or the intuitions of a nurse about an infant, than to trust the intuitions of a trader about a stock. We can confidently expect that a detailed study of how professionals think is more likely to reveal useful predictive cues in the former cases than in the latter.
This is a line of reasoning that has haunted me for some time. Is intuitive expertise possible in stock picking? The answer, at least according to Kahneman and Klein, is that it isn’t. Which then begs the question: what sorts of expertise exists in the domain of public equity investing? If intuition is to be distrusted in investing, what else does such expertise consist of?
I don’t yet have good answers to this question, but I have a better understanding of kind and wicked learning environments — the sorts of environments that Kahneman and Klein argue is so essential to the development of intuitive expertise. This is what we’re going to discuss today.
My original assumption was that Hogarth’s work informed Kahneman and Klein’s view, and that environments that provide adequately valid cues (kindness) and environments that do not provide such cues (wickedness) are simply immutable properties of the practitioner’s operating environment.
But I turned out to be wrong. Hogarth’s arguments were more nuanced than I’d originally anticipated. When you read Educating Intuition, you’d quickly come to realise that Hogarth was making an argument about the relationship between kinds of learning structures and the development of intuitive expertise: intuitions that arose in wicked learning structures should be mistrusted, whereas expert intuitions that were built within kind learning structures may be regarded as valid. Most importantly, though, Hogarth wrote that you may influence the validity of your intuitions — and the way that you do so is by picking the sorts of learning environments that you develop your expertise in.
In other words, kind learning structures and wicked learning structures are orthogonal to the kindness or wickedness of an operating domain. Investing may be a wicked domain, but you may construct kinder learning environments to scaffold investing expertise. And medicine may well be a kind learning domain (in that there are valid causal and statistical cues for disease; albeit with huge amounts of variability) — but you may find yourself in a wicked learning environment as a practicing doctor. In fact, Hogarth gives an example of exactly this in his book:
Consider physicians working in a hospital emergency room — a classic case of a wicked learning environment. Rarely do emergency room staff see the outcome of treatment. In cases of minor injury or illness, follow-up care is provided by the patient's own physician after discharge. In cases of more serious injury or illness, patients are transferred to a different ward for further evaluation and follow-up care. Emergency room staff therefore cannot rely on long-term feedback; they can observe only what happens in the short term. They also have few opportunities to experiment and often no way of knowing whether the task at hand is lenient (many conditions can be treated with antibiotics) or exacting (shortness of breath is symptomatic of many conditions, some minor, some potentially life threatening).
How do you identify a domain as kind or wicked? Hogarth give us some clues. In Educating Intuition, he proposes the following series of questions when assessing your own intuitions:
- Compare your learning structure with known examples. Is it, for example, like that of the professional tennis player (kind) or the emergency room physician (wicked)?
- How many times have you made this kind of judgment or decision in the past? Do you really know what your track record is? Remember that confidence in intuition can be a two-edged sword. Unless something makes us explicitly aware of past errors, having done things many times in the past can make us quite confident. But this confidence may not be justified.
- What kind of feedback have you received in the past?
- Has this been clear, that is, unambiguous? A criterion such as relative success in a job, for instance, could be quite vague.
- How immediate was the feedback? In other words, did you receive feedback on your judgment or action soon afterward?
- Was feedback compromised in any way? For example, could it have been biased by self-fulfilling prophecies or other actions?
- What role has luck played in past situations of this type?
- Is this important? If it is, it will be worthwhile trying to analyse it in some detail. Do not rely on intuition alone.
These questions may be inverted to produce recommendations for finding or creating kind learning environments for yourself. For instance:
- Structure your environment so you may receive clear feedback. That is: ensure that you have a unambiguous criterion for evaluating if your action was good or bad.
- If feedback is delayed, find ways to capture the moment in time when you made a decision, so that you may review it later when feedback is made available.
- Make sure feedback exists. (In the emergency room situation, this is difficult — you’ll probably have to do some systemic change where the outcomes of cases are forwarded to ER doctors for review, and schedules are changed to accommodate such reviews.)
- Check for compromise in the outcomes of cases. Compromise here means that results are subjected to some sort of self-fulfilling prophecy, where the decision to pick one thing over another results in that thing being successful. You’ll have to apply a fair bit of analytical rigour to consider possible counter-factual narratives.
- Set up methods for checking old cases, in order to disentangle (however partially) the effects of skill and luck.
- Finally, it is probably necessary to hold conclusions tentatively and loosely, subject to future revision — especially when partial disentanglement is all that is possible.
Recall that CFT is a learning theory that emerged from work done on accelerating medical expertise. When seen in this light, CFT learning systems are simply an attempt to provide doctors with kinder learning structures than if they were forced to accumulate cases through real world experience.
And so here’s my point: ill-structuredness is a separate property from the kindness or wickedness of a domain. It is totally possible to operate in a well-structured domain but within a wicked learning environment (where feedback is — for some godforsaken reason — withheld from you), or in an ill-structured domain but within a kinder learning environment (where it is relatively easy to track the outcome of decisions on highly variable concepts over long periods of time).
Investing is arguably more ill-structured than business — concepts have larger variability depending on the industry and sector you are looking at; longer-term industry patterns, market participants, and market structure all tend to change over a period of years. But the inherent wickedness of stock picking may be ameliorated somewhat by adopting best practices — it’s no accident, for instance, that decision journaling is more widespread amongst investors than it is amongst the broader population, and also not so surprising that both quantitative and qualitative case studies are more widespread in institutional investing. These practices help create a kinder learning environment than is immediately available in the markets.
Let’s wrap up. What have we covered today? We’ve been talking about CFT, a learning theory with interesting recommendations for building expertise in all sorts of ill-structured, real world domains. We discussed a handful of caveats:
- First, that CFT’s recommendations are mostly suitable for ill-structured domains. We examined how inefficient they are when used in well-structured domains.
- Second, we discussed how skill domains exist on a spectrum from well-structured to ill-structured. Some domains, like second language learning, occupy a space that’s in between. Learning in such domains probably demands a combination of learning both cases and concepts.
- Third, that ill-structured domains aren’t necessarily wicked, and that wicked learning structures may exist in all domains. I talked to a reader recently who told me that CFT gave him pointers on learning better in wicked domains. I said that I felt uncomfortable with this characterisation, and that I would dig deeper into the literature to see what they said. In the end, the explanation that most satisfies me is that CFT gives you a set of guidelines for learning under conditions of ill-structuredness, but it’s still up to you to seek out kind learning environments, or to create those kind learning environments for yourself.
For what it’s worth, I found this distinction empowering — it implies that it doesn’t matter what kinds of domains you operate in, or how ill-structured or wicked they are. With effort, you’re still able to construct kinder learning environments for yourself. If that’s not hopeful, I don’t know what is; I hope you’ll find the idea empowering too.
This is Part 4 of the Learning in Ill-Structured Domains Series. Read the next part here.
Originally published , last updated .
This article is part of the Expertise Acceleration topic cluster. Read more from this topic here→