This is Part 4 of a series on business expertise. Read Part 3 here.
Over the course of this series, we’ve talked a lot about Lia DiBello’s work on the mental model of business. It turns out, however, that DiBello is more well known for her training interventions — the bulk of her consulting work revolves around a training method she calls the ‘Strategic Rehearsal’.
The Strategic Rehearsal has its roots in her early academic work. When I was preparing for my interview with her, I traced the development of the technique back to her PhD, where it was called the ‘OpSim’ (under CUNY Professor Sylvia Scribner’s Laboratory for Cognitive Studies of Activity). This piece is a summary of every published Strategic Rehearsal that exists in the literature; I intend it as a resource for those of you who are looking for inspiration for your own training programs.
The good news (and bad news, I suppose) is that there aren’t that many published studies of the Strategic Rehearsal, probably due to proprietary arrangements with the companies involved. I’m limiting myself to published case studies so that I may link to source materials as we go along.
A note before we begin: as I’ve mentioned in the members forums, I’m not that excited about the Strategic Rehearsal, at least, not in terms of putting it to practice. As a training format, it is incredibly expensive to design and run … and, as you’ll soon see, I suspect there is a non-trivial amount of tacit expertise involved in creating the rehearsals to begin with. This means that it is unlikely I would be able to use the Strategic Rehearsal as a training technique for the organisations I am involved with.
What I’m interested in is what the Strategic Rehearsal represents. In 2016 the Department of Defence commissioned a report on accelerated expertise training programs. The way I understood it, the DoD had noticed that a number of Cognitive Systems Engineering scientists and Naturalistic Decision Making researchers were creating effective, accelerated training programs in both industry and military contexts. They wanted to know how these researchers were able to do so. As a result of her work with the OpSim and later the Strategic Rehearsal, DiBello was invited to become one of the authors; that report was eventually published as Accelerated Expertise.
Accelerated Expertise is essentially divided into three parts. The first part is a survey of the entire expertise literature circa 2016. The second part outlines the two learning theories that underpin the successful accelerated training programs these researchers have implemented in the field. The third identifies remaining holes in the empirical research base and proposes an ambitious research program to fill in those holes.
To me, what the Strategic Rehearsal represents is therefore less ‘here’s an awesome technique that we should study in order to use!’ and more ‘this is one successful, real world application of the learning theories outlined in Accelerated Expertise; the useful thing to study is the theory and principles itself.’ Mostly because I cannot see myself designing a rehearsal in my life.
(Not everyone agrees, though — as cognitive psychologist Joel Chan puts it to me: “I’m most excited about digging into her concrete techniques (they’re sort of instantiated theories to me) and the tacit knowledge she might try to articulate about how to do them well (…) as a HCI/systems/design person, I’m hesitant to abstract completely away from particulars of an artefact/technique.” Chan believes the rehearsal is worth studying to figure out why it works — and I think that’s a valid response as well.)
At any rate — you now know where I’m coming from. Keep this bias in mind as you read the strategic rehearsals below; I’ve written this collection from the perspective that it is the underlying principles that are interesting, and have focused on those above all.
Table of Contents
- Air Brake Remanufacturing (1997)
- Midwest Foundry (2000)
- NYCTA Bus Maintainers (2008)
- Biotech Firm (2009)
- Rio Tinto Miner Safety (2018)
- Maxx Appliances (2019)
- Exploratory Mining Simulation (2021)
- Features of a Strategic Rehearsal
Air Brake Remanufacturing (1997)
The first published instance of the OpSim is probably DiBello’s engagement with a large air brake remanufacturing facility, one that I last wrote about in The Importance of Cognitive Agility.
That study was about the implementation and adoption of a Material Requirements Planning system amongst workers at a remanufacturing plant. The workers consisted of Air Brake Maintainers (unionised mechanics), Supervisors (salaried and non-union managers), and Analysts (a general office position for those involved with planning and special projects). They came from a variety of backgrounds and — this being 1996 — had very little prior computer experience.
The OpSim was a manufacturing game. DiBello got the workers to ‘manufacture’ three models of an origami starship, each with common and unique parts, purchased from ‘suppliers’, stored in ‘inventory’, and pushed to ‘customers’. The workers were told to run their production lines however they wanted; their only goals were to reduce inventory and maximise profits by the end of the game.
The first run of the game was a disaster:
During the first morning of the first day, participants were allowed to run their factory according to any organisational scheme they wished. This invariably failed to achieve MRP goals (low inventory, increasing cash flow) and by the end of the first morning, most teams of participants were bankrupt, were unable to deliver, or began arguing with such intensity that the game had to be stopped.
After the first run was completed, workers were guided through a reflection exercise:
When this occurred, the game was paused and participants filled out a number of forms that helped them examine their patterns of decision making, evaluate their assets and losses, and reconstruct what had happened. These results were then compared by the participants with an MRP ideal of purchasing, production, and cash flow. After this phase, the participants were facilitated in the construction of a manual MRP system and introduced through various activities to its logic and overall functioning. Once they had a fully built and implemented ‘system’, they played the game again with the same customer orders and budget, but with very different results.
Note the phrase ‘facilitated in the construction of a manual MRP system’. The term ‘construction’ here is key — the idea that DiBello was investigating was this Piagetian notion that we only learn what we work out for ourselves, built on top of what we already know. I last wrote about this theory of learning when reflecting on Seymour Papert’s work — Papert was Piaget’s star student, and the person who pushed constructionist ideas of education the furthest after Piaget’s death.
It’s also worth noting that this study was really about the adoption of complex enterprise planning software, not specifically about achieving better business outcomes. DiBello’s focus here was firmly on the effectiveness of the constructive ‘teaching’ in between Run 1 and Run 2:
It appears that ‘constructive’ activities permitted individuals to reorganise the implicit mental models driving the decision process. As indicated, during the first morning of the workshops, the teams of participants could ‘run’ their factory in any way they chose. Without exception, under this kind of pressure, all groups ‘defaulted’ to the strategies they normally used at work. Comparing the performance in the workshops (as indicated by the cash and material flow patterns as well as the participants’ notes and inventory control records) with work history indicates a strong correspondence between current work practices and decisions under pressure. For example, mechanics almost always delivered a high-quality product on time and thereby managed to stay financially above the water by ensuring income. However, they often did so by buying finished assemblies (as opposed to raw materials) at great cost, by accelerating production (which has the real-world equivalent in ‘overtime’) and by overbuying all materials. Thus, they sacrificed profits.
In contrast, Analysts and Supervisors were often not successful at meeting customer orders but they were very hesitant to overspend; often they could not meet orders because they had waited too long to decide to purchase the necessary materials. It is important to note that individuals resorted to their default strategies even when explicitly instructed to operate differently and when they were aware that the goal of the game was to make the most profit and buy the least material.
As indicated, during the workshops’ first pause, participants evaluated their strategies and decisions using various tools. During the discussions that followed the self-evaluation, participants began to reflect on these default strategies and examined how a different approach might have accomplished their goals. It was only at this point that participants became aware of the assumptions guiding their decisions under pressure and the attendant results. We have come to call this the ‘deconstruction’ phase and the beginning of ‘reorganisation’. During the MRP-oriented ‘constructive’ activities that followed in the next day and a half, participants were introduced to MRP concepts as they continued a pattern of inventing a solution using their intuitive understanding of manufacturing, noticing its mismatch with their goals, reordering what they had done, and comparing it again to their goals. At the end of this process, all participants arrived at the same solution but, it is important to note, they had all gotten there via different paths and had begun the process through sometimes radically different entries.
Even in this initial study you can see all the elements that characterise a Strategic Rehearsal: the physical setup that triggers ‘default schemas of cognition’, the fixed goals that are projected overhead for all players to see, the accelerated nature of the simulation, the two runs with a reflective session in between, and finally a ‘cognitive probe’ done before and after the intervention to evaluate training effectiveness.
Source: Exploring The Relationship Between Activity and the Development of Expertise: Paradigm Shifts and Decision Defaults, in Zsambok & Klein, Naturalistic Decision Making.
Midwest Foundry (2000)
The second published instance of a strategic rehearsal is the one involving the Midwest Foundry Company (MFC). A more detailed version is available in my interview with DiBello; the events here occurred circa 2000.
I shall take the story told in The Oxford Handbook of Expertise, since it is the most concise:
The Midwest Foundry Company (MFC) is an old foundry that makes all sorts of iron castings, including small machine parts and large-scale castings such as cast iron engine blocks for freight train locomotives. However, over the past several decades, the foundry business has changed drastically. Many items once cast in iron are now fabricated using other means, and small castings are outsourced offshore at greatly reduced cost. However, there is still modest demand for domestically made very large (multi-ton) castings, which are normally not made in large quantities. MFC had facilities capable of making castings weighing as much as 30,000 pounds, with their average casting being 5,000 pounds.
When sales in 2000 were down 11 percent—the general perception was that the company was losing money because it was losing sales. As a result, the owner and the management entered into increasingly risky agreements in order to attempt to retain customer business. These were viewed as marketing programs designed to secure additional business. However, many times prices charged to desirable customers were below cost of production for MFC. As such, the more castings they made, the more money they lost.
MFC’s real opportunity resided in their close proximity to their customers and their ability to make very large castings, but they needed to look at the opportunity differently than they had been. They needed to offer greater value rather than lower prices. Customers would pay a premium for something they need quickly and which is hard to get. Large castings are often used in other products that are not bought in large quantities but which, when needed quickly, are highly profitable for the seller. Therefore, price cuts were not necessary for items customers are desperate to have.
DiBello’s strategic rehearsal contained all the elements from the air brake remanufacturing intervention: two days, one exercise on each day, with a physical simulation designed to feel like the actual art of mould making, pouring and finishing (they used a polymer sand, which when mixed with water would make temporary moulds for plaster of Paris castings). The moulds fell apart within 30-45 minutes, which reflected real world sand moulds that decomposed within days.
Before they ran the rehearsal, however, they did an extraction of the default mental model, to identify what was missing from their understanding of the work domain:
Before designing the mock work environment, we needed to understand what kinds of thinking supported their current practices. We asked the workers to develop an as is map of their process, outlining how they see the workflow from pattern to delivery, and subsequently develop a to be map.
During the rehearsal it emerged that their as is map did not include on-time delivery, and their to be map seemed to be an extension of the current way of thinking—they simply created new ways to push more product through the pipeline, without realising the new bottlenecks that this would create. In addition, for the mould makers, cleaning and shipping were not part of their process maps. Activities not involved in actually making the casting were not the object of their thinking and decision making. Therefore, entire parts of the process, such as handing off the job, trucking the unfinished casting to the cleaning facility, and storing were not represented on their maps and were not objects of their attention. We needed to get them to think about these missing things.
If their old ways of thinking had emerged in response to adapting to one environment, we needed to create an environment with new goals that were highly visible. As such, the rehearsal design began with a set of outcomes to which they would be held accountable:
1. Customer orders must be shipped on time; anything late had to still be delivered, but there would be no payment for it;
2. A lower cost per item budget;
3. Reduce scrap rate goals; and
4. A specific revenue goal with specific profit margin that had to be met by the game’s end, with reasonable interim milestones along the way showing progress.
The rehearsal went much like the remanufacturing rehearsal DiBello conducted a few years before: under pressure, the workers defaulted back to their default work ‘schemas’. They produced beautiful castings, but always late. They began to fail, badly.
While making moulds was easy for them, in the context of new requirements the task became more complicated. For example, there are about eight steps to making most castings. If you are late with step 2, you are already too late, even if the casting is not yet due to the customer. Thinking about the lateness of a casting by step 2 was not part of their thinking. Time had not been thought about in that way before in the context of their work. To develop this kind of thinking, we gave them tools offering visibility into the effect of accumulating lateness. One tool was a MRP (material requirements planning systems)-generated list of internal deadlines (by customer order) for internal customers (i.e., the eight steps in the process) such as making the cores, the pouring room, or finishing facility, which allowed to them see that delay in one process had a domino effect on other parts of the process, even if it is weeks ahead of the planned ship date. We required that the MRP router (a printed form with all the steps and interim due dates) travel with the job (pattern, mould, and casting) and each person had to check off their step and when they did it. The due date (or game period in this case) was shown for each step, not simply the due date at step 8. Thus, at every hand-off, they could see the accumulating lateness (emphasis added). In addition, the customers (played by staff facilitators) did not always accept orders that were late and certainly did not pay for them. MFC had had a MRP system for nearly four years, but they had never used it except to track customer information, such as shipping addresses, customer item numbers, and sales contacts.
By the end of the first day, the facilitators had stayed in the role of unhappy customers for the majority of the exercise, and the workers were distraught:
(…) we tracked their performance and projected charts using an LCD projector. The projected charts were updated every few minutes. Even though they could see where they were headed, they could not come up with a solution. In order to get the customers to take the castings, they cut prices below cost and tried to make up the losses with volume. Even though this group had developed a careful to-be plan and process map, this was entirely abandoned during the rehearsal. The phones keep ringing and the suppliers kept sending bills.
Before the second day began, the facilitators allowed the workers to meet privately in order to devise a new approach. They continued their discussion a few hours before the second simulation started. DiBello includes this snippet of conversation in The Oxford Handbook:
D: Okay, but when you’re done with making that, you can’t just say “okay I did my part” and make a little pile at your elbow. I am waiting for you to be done with that so I can do my piece. You have to let me know it’s ready or give it to me, because if you get it to me later than I need it, it’s late, period.
T: Right, and the whole team doesn’t get paid.
L: Yes, we don’t get paid. Everybody got that! You don’t move your stuff, we don’t get paid!!!!! So maybe we should move you guys to be closer together and come up with a way to signal that you’re done.
B: Listen up you guys. I just got the customer to agree to a higher price with a shorter lead time. Can we do that? Let’s look at the routing and see if we need all the time on there. (One of them had gone to the facilitators and asked if they would be paid a premium if they were early).
T: Has anybody figured out the break-even by piece? Are we charging enough? We need to look at the prices; the more stuff we made yesterday, the more money we lost.
The workers were then allowed to run the simulation a second time, this time with a rearranged workplace and better use of the MRP router. DiBello then notes:
It should strike the reader that long-term experience of the workers has not changed, but their thinking is changing, both individually and collectively. In fact, it is their experience that makes the new level of expertise possible, even though before, it was the very thing holding them back. By rearranging the context in which their expertise is deployed, new goals will select ways of thinking and develop capabilities that make meeting those goals possible. The design of the rehearsal only focused the participants on accountability for outcomes, not on the best way to get there (emphasis added). This stimulated participants to begin to find new ways of thinking about the same things.
By the end of day two, DiBello notes that the workers had designed a ‘pull’ manufacturing system, similar to kanban, instead of a ‘push’ system. After nine months, the MFC was on time nearly 82% of the time, and returned to profitability. They reduced their scrap rate from 13% to 9%. They focused on premium pricing and on-time delivery and gradually retired the commodity products in their lineup.
Why did it work so well? DiBello argues that the foundry workers had all the expertise needed to accomplish this change. She didn’t really teach them anything — she merely surfaced their default work schemas, disequilibreated them (a Piagetian term meaning she made them lose faith in their existing mental models) and then she … reorganised those models by giving them a different workplace context.
But so much for the theory. What happened next?
A few months later, the company confronted a crisis.
The owner was involved in a lawsuit with the bank; he was accused of using bank loans secured for capital improvements to make acquisitions of other businesses. The bank took possession of the company and the owner suddenly passed away. This was very discouraging news since the workforce had made considerable progress in understanding the key to profitability in today’s market. Although the plant never actually shut down, the workforce struggled to meet commitments. They found it hard to pay suppliers with assets being seized by the bank.
After trying to negotiate with the bank, some of the managers who had participated in the rehearsal sought out and found a financial backer. This backer bought the company on an “assets only” basis (he did not assume the debt), which allowed the workforce to continue with their program of improvement while the investor acted largely as a silent partner. The idea was that they would repay him with interest from the profits.
The MFC survived, but only by slowly paying off the debt with profits from the business. DiBello writes in The Handbook about their two-year follow-up with the company:
On our return visit after two years, most of the same people we had worked with were still there. Orders were being pulled through the system in an orderly fashion, on time, at a low cost, with little scrap. They had achieved 100 percent on time to the customer and had shortened their lead-time from six weeks to two weeks and negotiated premium prices from customers for shorter lead times. They had reduced their scrap rate down to 2.9 percent, well below the 6 percent industry average. The entire customer service department was eliminated, profits were consistent, and for a foundry, the margins were higher than is typical.
We found that they were still using a version of tools developed in the rehearsal, but had gone beyond them, innovating new technologies. This was remarkable for a workforce that had been computer illiterate two years before. As the industry changed again, the workforce modified the information tools to support better decisions. Each team of workers gets information each morning with all orders and all offsets, and a detailed version of accountabilities for the whole company. The larger goals and complexities of the entire process were made transparent to workers in all parts of the process. In other words, every worker has visibility of his or her work in the context of the whole business and all workers have access to the tracking technologies.
The MFC is owned by its workers, and still running today.
Source: Expertise in Business, The Oxford Handbook of Expertise.
NYCTA Bus Maintainers (2008)
The New York City Transit Authority runs a fleet of over 4000 buses and 8000 subway cars. I’ll give you the setup:
The Bus Maintainers on the shop floor of the New York City Transit Authority (NYCTA) were in the middle of a complex organizational restructuring. At the time, the NYCTA was about to experience a 10% increase in ridership and had been informed of a $300 Million budget cut in their operating and maintenance divisions. They had also bought a new fleet of buses from a new vendor (a procurement process that literally takes up to 5 years) but when the buses arrived, they did not meet standards and had to be taken out of service. A prior vendor was consulted, but could not replenish the fleet to replace the buses that were due to be taken out of service. Ultimately, the new fleet never arrived, so the maintainers had to deal with the 10% increase in ridership with no new fleet. Increased ridership meant that the maintainers had to do more with less. In other words, they were dealing with an increase in wear and tear on the buses, while charged with the task of increasing what is called the ‘‘mean distance between failure’’ (MDBF)—a key metric in assessing the ‘‘health’’ of the bus and the efficiency of repairs or proactive maintenance plans. Higher MDBFs also translates into fewer days in the shop and more days in revenue-earning service, translating to a better bottom line for the NYCTA.
In addition to all of this, NYCTA management wanted to implement a ‘‘cycle based’’ preventative maintenance system involving a new complex IT system, replacing the standard ‘‘reactive’’ system already in place. Given the disappointing track record of IT implementation among so-called ‘‘low level’’ workers, such as the Bus Maintainers of the NYCTA, management was nervous. The Workplace Technologies Research Group (WTRG) had been called upon to address this particular anxiety, which is common among IT implementation efforts: How do we design an efficient, yet complex IT system that is useful for workers with little computer skills training and whose existing culture of practice is generally resistant to such efforts? Further, how do we deploy it work with their considerable skills, which may be critical to the success of maintenance activity but which are not captured in systems per se?
You’ll notice that many of these early interventions are technological in nature. This isn’t an aberration — DiBello’s PhD supervisor, Professor Sylvia Scribner specialised in workplace cognition, and DiBello’s early work was almost exclusively focused on the (often difficult) adoption of new workplace technologies.
This NYCTA intervention was no different:
For many years, NYCTA management wanted to implement a centralized ‘‘cycle based’’ maintenance system. Manual systems proved unwieldy, given the size of the fleet (over 4,000 buses and 8,000 subway cars), and it was widely acknowledged that early information technologies failed for many of same reasons cited earlier in this paper.
1. The information needed to make them work had to be extremely accurate at the right level of detail. Ideally the information should be inputted by the mechanic him or herself.
2. Efforts to train mechanics on computer use had not been successful historically. In general, it was widely acknowledged that this population did not contain, by and large, ‘‘classroom learners’’. At New York City Transit, many of the workers did not speak English as a first language (about 80%) and had virtually no keyboard training.
3. System sabotage. Front line workers are usually threatened by information systems on the shop floor, often seeing them as ‘‘time and motion’’ studies in PC form. Such attitudes and the relative vulnerability of computer systems led to widespread system sabotage or damage to expensive computer equipment.
So what did DiBello do? The first step was to do an initial extraction of the bus maintainer’s mental models. This was difficult, as management had differing ideas of what the bus maintainers did. DiBello used a variation of Gary Klein’s Cognitive Decision Method (one of the earliest skill extraction techniques developed within the field of Naturalistic Decision Making):
Our method involved the following steps:
1. Identify the strategies and practices associated with each domain that make sense only within the ‘‘world view’’ of that domain. E.g., most workplaces have more than one theory of the work being done, and occasionally these compete. Our field work showed us that maintenance is both reactive and proactive, depending on the goals considered most important and the available resources. In NYCTA, the lack of good data for proactive planning and the ‘‘make service at all costs’’ emphasis in the organization tended to favor reactive maintenance. However, proactive practices were also in play, when time and information permitted.
2. Identify behaviors associated with these strategies in the workplace in which we are doing the research. Simply put, we looked at how the different kinds of thinking manifested itself in day-to-day decisions.
3. Design a meaningful ‘‘problem’’ situation into a ‘‘cognitive task’’ that can be solved using the strategies and behaviors from either domain, or a mix of both during a short interview.
4. Design a problem situation that is similar to that in #3, but which is more abstract and generic than the site specific version.
5. Develop a scoring form that permits a coder to check off the strategies/behaviors easily and calculate the proportion of the strategies used from each domain.
For this cognitive probe, DiBello et al constructed two basic tasks:
The first task was an ‘‘active’’ task; given a pile of work orders, we asked the interviewee to look them over and then make five piles for each day of the workweek. In other words, schedule the work.
And the second:
The second task required the interviewee to interpret information in bus repair histories. Again a bicycle repair history and one for ‘‘machine T’’ was also included. A similar set of strategies (only for interpreting data) as those shown above was used to code the protocol. Photographs were taken of the interviewees’ piles and any drawings or writing and all talking and ‘‘thinking aloud’’ was audio taped.
From these two tasks DiBello’s team learnt that around 60% of the bus maintenance strategies were reactive and less than 40% were proactive. This went against what management believed — that the frontline maintainers were only doing reactive work, and that workers weren’t already doing preventative work when possible.
DiBello and her team decided to construct a three-part simulation:
Teams of eight participants each were asked to ‘‘run a depot’’ of 40 plastic buses with relatively complex interior components. Ultimately, 3,000 people over 13 months participated in the exercise across the NYTCA bus depots. Two locations would run the exercise at a time for 2–3 weeks and then we would move on to the next two locations. The process lasted 13 months. Each depot had between 200 and 300 people. For the OpSim, participants were broken up into teams of eight according to their shift. For example, if the day shift had 80 people, there were 5 sessions on the day shift with 16 participants running through the exercise at a time, set up in two competing teams of 8 participants each.
The goals were to maintain 32 buses in service at all times (limiting the number out of service to eight), order all the materials (within a budget) needed for doing so and evaluate daily operator reports (each ‘‘day’’ being 20 min) that might indicate potential problems (e.g., noisy engine). The activity was ‘‘rigged’’ so that the only way to meet these goals was to predict what was due to break next. The breakdown patterns of all components followed time/ mileage cycle rules and were pre-calculated using a computer. The toys were actually ‘‘broken’’ according to this pattern. The participants were given adequate tools to predict and calculate this breakdown, (printouts of every bus’ repair history among other things) but were given other tools as well, including those similar to those used to do ‘‘reactive’’ maintenance.
Our trainers also played a role. One acted as ‘‘dispatcher’’ regularly demanding buses to satisfy routes while the other acted as a parts vendor and an FTA (Federal Transit Administration) inspector, looking for safety violations or ‘‘abuses of public funding’’ such as overspending or cannibalizing. Close examination of the use the participants made of the tools offered pretty much approximated their work history. People tended to construct a solution to even a novel problem that fit with their experience, even when explicitly instructed to avoid doing so. In fact, the participants were rarely aware they are replicating their normal methods.
Rather than interfere with this tendency, the trainers allowed the participants to ‘‘wing it’’, while carefully documenting the cash flow, labor flow, inventory acquisitions and the number and type of on-the-road failures that result from failing to predict problems. Meanwhile, heavy fines are levied for expensive ‘‘reactive’’ problem solving strategies, such as ‘‘cannibalizing’’ an entire bus for a few cheap parts that will get other buses back on the road. As the activity progresses, participants are continually shown the financial consequences of their decision making patterns and asked ‘‘what they were thinking’’ by the vendors/ inspectors and dispatchers. By the end of the first day, the ‘‘depot’’ is in crisis and the participants are realizing their budget is being expended to react to mounting problems. The activities are stopped and the team is sent back to work or to lunch.
The second part was a facilitation workshop. DiBello and her team guided the workers in a reflection exercise over their first run performance. They then facilitated the workers in the construction of a manual scheduled maintenance system (with pencil and paper) for the entire bus fleet. After doing this, the maintainers were asked to enter data on an actual test region in MIDAS (the centralised maintenance planning software that NYCTA had built) and created and assigned new work orders according to this schedule.
In the third part of the workshop, they were asked to repeat their bus depot simulation using the MIDAS schedule and see the difference in profits and ease of workflow. This, time, the workers completed the simulation effortlessly.
Before ending the workshop, the researchers got them to do one last thing:
The last activity of the workshop involves entering the data on work orders (paying attention to detailing the components, defects and symptoms involved) and closing out both work orders and work assignment sheets. At this point, participants also learn how to get various reports that they now realize they will want, such as a 30-day history on a bus. After operating as MIDAS and then with MIDAS, participants navigate through the actual system more easily, know what to look for and ask informed questions. Even computer illiterate individuals show little hesitation when exploring the system.
The entire exercise was conducted over 13 months with over 3000 bus maintainers. DiBello notes:
As indicated above, about 80% were not native English speakers and fewer than 20% were computer literate. Many mid-career individuals had not completed high school. None wanted to attend the training and most were resistant to the idea of having to do their own data entry.
Despite these features of the trainees, they mastered the system at record speed: Rather than requiring the expected 12 months for implementation, the hourly staff reached independence with the system in 2 weeks and line super- visors (who do more) managed in 6 weeks. The one exception was a location that received classroom training but no OpSim intervention. After 8 months, the implementation was being declared a failure.
What were the long-lasting effects? Well:
The overall average (MBDF for the whole fleet) rose with the number of depots that had completed their MIDAS implementation and hence were having a greater first pass yield on repairs. The savings in field supervisor time (handling the return of broken down buses) is estimated to be 208,000h times a fully loaded hourly rate of $70, or $14,560,000. These numbers represent the financial benefits that were incurred even before there were enough data collected to do the kind of trend analysis needed for true preventive replacement based on life cycles. That analysis was just beginning about 2 years after the system was fully implemented.
For the transit industry, the MIDAS project has been the first successful front line deployment of a Centralized Maintenance Management System and, one of most successful and enduring implementation of a CMMS in general. The interesting portion of the project from our point of view is that when the technology was implemented as a tool for ‘‘experts’’, it extended the existing content knowledge of the workforce into a form that could be used for an aggressive change in the way business was done.
Finally, DiBello notes with some interest that within a short period of time, mechanics started using MIDAS in ways that nobody expected, in order to augment their distributed knowledge of the fleet. She writes:
… mechanics increased their use of an ‘‘optional’’ feature of the system, the free-form notes attached to each work record. Mechanics not only entered notes with increasing frequency, but read the notes of others as well. As time went on, these system notes became increasingly in the private language of mechanics. As the mechanics grew more comfortable with the system, it became harder for us to know what they were doing with it. In other words, they grew beyond us in their understanding of what the data were saying and the best way to enter it.
Below is an excerpt from the ‘‘notes’’ section of a work order at New York City Transit:
Worked on 7016, which came from ENY minus the following items: one entrance door partition, one station upwright and grabrail, one dome light partition cover and front dest sign lock. Remove dest compart locks from bus 7033—which is waiting for other parts—to meet req. All other items listed were obtain from spare buses at yard. Tap-out damage Riv-nuts installed new ones on same. Interior close to be continue.
There are two striking features of this passage. The first is the admission of ‘‘cannibalism’’, (stealing parts from one bus to get another into service), a practice that could have led to dismissal before MIDAS was implemented. Using MIDAS, mechanics soon realized that indicating parts shortages in the components fields helped MIDAS correct parts ordering forecasts, making cannibalizing unnecessary. Telling other mechanics where the stolen parts came from helped them address missing parts problems in the cannibalized buses later. Other notes helped the mechanic on the next shift begin where the other left off. The other striking feature is that we cannot decipher very clearly what is going on. In other words, the notes are not useful to us non-mechanics. This trend became more pronounced as the system produced more profound financial benefits. What has happened here is that the system has become a tool for the mechanics, and perhaps this has been the problem all along with failed technologies.
Biotech Firm (2009)
This company is probably Invitrogen, but the company’s name is never mentioned explicitly in the paper. I’m mostly guessing this fact from the funding source, and from the authors (one author, Marie Struttman, was from Invitrogen, Inc).
You know the drill. Here’s the setup:
Our site is a biological manufacturing company that makes proteins, bacteria, and other biological materials used for scientific research and drug testing. Their “brand promise” is to make scientific work faster and easier for scientists by eliminating steps in their process. Many of the products they make would have to be made by biologists working in labs as part of doing an experiment on something else. The idea is to make those routine steps into standard materials available for purchase. This process requires that they make labor intensive but necessary materials on a grand scale.
The company grew by acquiring literally scores of much smaller companies and their workers. Most of these start-ups comprised small teams of biologists who formulated unique products (such as proteins made by genetically engineered e coli), patented the process, and then manufactured the products in small batches. The acquisition strategy assumed that once a “recipe” is perfected, a technician who is not a biologist could be trained to follow the recipe and make the materials in larger batches. This would reduce costs, transform the material into a true product, and increase margins for the parent company while avoiding the time and money needed to formulate new recipes from scratch. More to the point, it would create a $3 billion business supplying scientists with material they need anyway. However, the collection of smaller companies under one roof brought in the informal small-group cultures and inefficiencies of the small labs. When we first conducted interviews at the company, we heard managers tell us, “We have about 25 small companies on one shop floor, and they are not working as one company.” The net effect was a kind of scaling crisis that was inadvertently hidden by metrics normally used in large businesses. This led to a number of serious production problems that could not be nailed down.
The main problem with this company was that it had large number of back orders. This was by far the biggest complaint from customers, and the company had already run a number of improvement efforts, diagnostic committee meetings, and in-house ‘studies’, all of which proved inconclusive. Worse, in interviews with production employees, DiBello et al discovered that the employees uniformly believed that this complaint was ‘overblown’:
They claimed that standard reports showed only about 2% of the products on back order at any given time. However, the company has about 5,000 products in their catalogue, of which 3,500 were active SKUs. Of those SKUs only 198 were 95% of the total orders. When looked at that way, the number of customer orders and revenue dollars tied up on back order approached anywhere from 40% to 80% at any given time. This amounted to as much as $800,000 worth of customer orders per day on back order. When confronted with these data, both workers and managers were surprised. They then defended the back order numbers by claiming the products are the result of biological processes that cannot, by their nature, have a firm deadline. “Sometimes the bugs don’t grow and you have to wait or start over again.” If this were in fact true, the company had a serious problem; the products were needed by scientists at critical points in their work; they could not wait for back orders and were forced to go elsewhere when the site could not supply them on time. When confronted with this fact, the staff next countered that the forecast itself was flawed. They explained to us that the company had a kind of retail business model, shipping things ordered from a Web site, often using overnight delivery. This model assumed that products ordered were in stock. The employees told us the forecast indicating what customers would buy was “always wrong” and this explained the high percentage of back orders.
Well done. DiBello et al continue:
When we drilled down into this situation, it was clear that the forecast was in fact relatively accurate, based on customer buying patterns and commitments from customers for future purchases. Why, then, was the back order problem so huge? When we compared the forecast to shop floor production, it was clear that the production personnel largely ignored the forecast, “cherry picking” work orders rather than making things to schedule. Workers made items they genuinely believed would be needed. They thought they were working around the inaccuracy of the forecast. In fact, they were contributing to back orders.
Most important, our research also revealed that some individuals manage to get the right number of “bugs” to grow to the right volume, on time, when necessary. The people who managed to pull off this miracle were usually not highly trained biologists and certainly not in the management ranks. We began to suspect that implicit expertise had developed through experience but that it was activated in response to crises, not business as usual. More to the point, this ability—however activated—demonstrated that it was possible to be on time when making and selling biological products on a large scale.
Notice that the challenge was slightly different with this company, as compared to the the air brake remanufacturing plant, the Midwest Foundry, and the NYCTA bus maintainers. With those earlier rehearsals, the challenge was getting manufacturing workers to adopt complex Materials Resource Planning software, and therefore adopt lean manufacturing best practices.
With the biotech company, however, the challenge was in distributing knowledge of the problem to production employees, as well as spreading intuitive expertise from front-line technicians to management and other line supervisors. From the pre-intervention interview I excerpted above, DiBello and team had identified that the workers were fairly wedded to their mental models of the workplace. They had repeatedly found ways to reject evidence that the backorder problem was real. This was going to be tricky to fix.
Or was it?
Again, DiBello et al ran an OpSim, this time with coloured liquids standing in for bio-products:
… the business challenge in our project was threefold:
1. Reduce the high level of back orders.
2. Empower frontline workers to devise a solution while holding them accountable for the performance of the company.
3. Sustain the results and increase the chances that the solution would transfer back to real work.
To address these challenges, we designed a fast-paced OpSim exercise that replicated the shop floor’s major functional areas. We used an undeveloped section of the company’s facilities, building a “shop floor” within a 40 × 60 ft space. Using our software, we modeled the ideal financial profile of a company with growing sales and no missed shipments. Using a method of calculating the net present value of the company, we also derived a “tracking stock,” showing how high performance would translate into a rising stock price. At the start of the exercise, we simply told participants that in order to win, they had to meet all customer orders on time. We did not target or even specify the skills or means for greater responsiveness; rather we emphasized only the goals (on-time delivery, no missed orders, increasing volumes, and a revenue and profit goal) and challenged the groups to develop a solution. The only feedback they received was a dynamic dashboard showing the financial impact of their decisions and actions. This was projected on the wall from a laptop so the participants could see in a relatively “live” fashion how they were doing relative to the “ideal” on key factors such as the following:
1. Orders fulfilled
2. Cost of goods sold
3. Gross revenues
4. On-time status of work in process at all major stations.
5. On-time status of customer orders
Four groups of 20 to 25 people participated in the simulation exercise for 10 hr each. Each group got two chances (playing the game once a week for 2 weeks, for 5 hr each time) to achieve the target outcomes. In the 5-hr period, the team was given 1 hr to plan and organize their company and then had to complete “12 weeks” (equivalent to one fiscal quarter of business) in the remaining 4 hr. Therefore, it was conducted under a compressed time frame with each “week” lasting only about 20 min. All teams were competing with all other teams. The team with the solution that solved all problems and accomplished or exceeded the revenue, on-time delivery, and profit goals would be the “winner” and their solution would be implemented on the actual shop floor. As part of the project, senior management agreed to support the implementation of any changes that proved successful in an OpSim. In other words, the OpSim was seen as a kind of opportunity to invent, rehearse, and refine an operational approach to the goal. Success in the OpSim was seen as proof of a plan’s worthiness. Director-level management participated in the OpSim to experience the business value of emerging innovations. They played in roles that would be directly affected by the innovations: customers, research & development personnel trying to get new products launched in manufacturing, and the shipping department.
The first run resulted in failure, as expected. The facilitators had taken care to use the context of a simulation to remove the ‘excuse’ of a bad forecast — as all forecasts in the OpSim matched customer orders perfectly. And yet the workers reproduced nearly the exact same results: a 40% back order rate, resulting in as much as $800,000 of customer orders on hold and at risk of being cancelled. When faced with this evidence, the workers had no choice but to admit that there was something very wrong with the way they were doing things. Participants were asked to reflect on what they might do differently. They then ran things a second time:
In the second round, after given a clean slate and a chance to retry the game, the groups were able to resolve the problems and failures they confronted in the first round. In fact, all groups exceeded the desired outcomes. In addition, they accelerated the launch of many new products by several game “months,” increasing revenues beyond those projected and causing the stock to spike as they beat out virtual competitors trying to launch similar offerings. The groups achieved these goals in innovative ways and developed solutions that they had not previously considered. In the second round, rather than working faster or following new procedures, they developed a greater understanding of manufacturing’s role in the total business. Rather than focus on local goals, they focused on tailoring their activities to higher level goals, such as revenues, profits, and the impact of these on stock price. Thinking this way led them to redesign the shop floor flow of material and information, minimizing product movement and centralizing some activities. Although there was a “winner,” all the teams found a similar solution to the back order problem and achieved similar results. In time, the winning team’s solution was implemented in real life.
Once again, DiBello highlights the fact that they haven’t taught the workers anything new:
From our experience, we think that OpSims accelerate learning because they elicit the implicit knowledge that the worker has to bring to the problem and at the same time select against non-workable strategies through experiences of failure (emphasis added). That is, participants in our interventions learn by “unlearning” their heuristics and reorganising their extensive content knowledge more than really learning something new.
A year later, the researchers checked back with the company to see if the simulation outcomes had taken:
When we returned to the company a year later, many of the solutions they developed in the simulation had been put into practice. They implemented the shop floor design and metrics that emerged from the winning team during the exercise. As in the exercise, the real-life metrics focused on the high-level financial impact of day-to-day decisions and actions (emphasis added). They had implemented a system to track back orders based on those products that had generated the most revenue, and the back orders (in dollars) were posted daily. In fact, the workers chose to use an LCD projector exactly like the one used in the OpSim to get “live” feedback. Each supervisor was rated on compliance to the forecast, also on a daily basis. In addition, they reorganized the structure of teams (both in the simulation and subsequently on the actual shop floor). For example, prior to the simulation each team responsible for different parts of the bio-manufacturing process was also responsible for their own vialing and packaging. Prior to the OpSim, there was no centralized vialing and packaging. During the OpSim, all teams realized the need for centralized vialing and packaging, and the best solution to this problem was generated within the OpSim and subsequently implemented on the shop floor, which contributed significantly to a drop-off in back orders.
I think it’s worth asking at this stage if the rehearsal exercise is powerful because it teaches employees the connection between their day-to-day activities and top-line metrics for the firm. I speak from experience when I say that, as a high-level manager, I had difficulty mapping some of the things we built to end-of-year financial results (“I guess this project cost us $50k to build, and we … never recouped our costs? But, eh, we made 800k in profits from other shit, I guess it was ok.”); I cannot imagine that a front-line technician or a factory worker has much sense of the financial impact of their work.
And so now I wonder if a huge part the Strategic Rehearsal is that it exposes employees to the real systems that underpin their company’s performance. If you have direct exposure to your company’s underlying mechanics, I find it very likely that you’ll evolve your mental models to fit the true goals of the system. And if you do this through a mandatory exercise, across all your employees? It’s not very surprising that you’d turn your company’s production around.
I’ll close this section with a few paragraphs from the authors on why they think the OpSim worked:
We have told a story of workers at a biological manufacturing plant and their success. The challenge now is to explain what caused their success (see Figure 3 for pictures). Was it the OpSim experience and its emphasis on manufacturing’s contribution to the total company? Was it the fact that the workers could implement the things they discovered in the OpSim? Did they enjoy continued success because of the sustaining metrics that were exported from the OpSim to the shop floor? Several challenges present themselves when doing research in naturalistic settings, with real companies where classical experimental methods are not possible. However, the results are suggestive. The fact that this type of simulation had a quick and dramatic impact suggests some things about learning and the nature of in situ cognition, particularly considering that changes did not occur until the teams attempted a second time, and considering all four teams failed the same way the first time, and the succeeded the same way the second time (emphasis added).
(…) We believe here that by the time they went through the simulation the second time a week later, they had found they had reorganized their content knowledge according to a new framework that was more adaptive to the goals at hand. In other words, just as the prior approach was assimilated almost reflexively the first time, the new approach was assimilated without effort the second time with marked success.
However, a Piagetian approach only takes us as far as understanding what might have happened within the individuals. It does not explain where the goal comes from and how changing goals and feedback can influence new models of thinking and acting. For Piaget, the prior approach would be as developmentally advanced as the one that replaced it. This was adaptive, for the most part, until the workplace structure and goals had changed. To address the role of changing context of development, if we look at the process in terms of Vygotsky’s activity theory, cognition is always developing in service of a leading goal or activity. In this analysis the zero back order goal was a leading activity in the Vygotskian sense (emphasis added). The socially constructed requirement acts as a context that constrains what develops in that it determines what is adaptive to the situation. In other words, Piaget’s functional invariants combined with activity theory’s notion of leading activities in a social space, best describe the underlying mechanisms we believe are at work in the OpSim.
The principle of “equilibration,” which describes the way in which humans harmoniously integrate prior knowledge into strategies for solving problems in novel situations, is central to Piaget’s approach. This Piagetian model involves what he terms the “functional invariants”— assimilation (applying existing schemas to familiar aspects of a novel situation); accommodation (incorporating novel information to modify existing schemas), which lead to an equilibration of schemas through the processes of disequilibration; and reorganization. Disequilibration describes the confrontation between existing schemas and incompatible feedback from the environment and from the existing community of practice within which the activity takes place (emphasis added). It is through the process of disequilibration that the strategies of assimilation and accommodation (the basis of learning and development) are activated. As such, disequilibrating existing mental models becomes a central feature of the accelerated learning dynamic within an OpSim.
In the end, it’s only human to adapt to what you can see.
Source: The Long Term Impact of Simulation Training on Changing Accountabilities in a Biotech Firm.
Rio Tinto Miner Safety (2018)
In a 2018 article in Psychology Today, Gary Klein tells the story of a Strategic Rehearsal with Rio Tinto miners:
WTRI had been brought in to put on some training for Rio Tinto — the training was tested at an exercise in a (virtual) underground mine. The training seemed fairly mundane and the miners didn’t see any reason to spend two whole days on it. They were highly experienced and knew their jobs well. They complained loudly about having to go through the WTRI training, and complied only because upper management insisted. (…)
The virtual world layout had been carefully designed to match the actual layout of the mine as it will likely be in 2025, so some of it was very familiar, and some of it was new, but part of a well-known plan. In fact, it was the future layout the miners were engaged in building in real life. So this was no cookie-cutter exercise. The miners felt that this was the real thing. (…)
Each trainee was alone in a room with a computer, wearing a headset and navigating the virtual world with a joystick. However, they were not alone in the virtual world. They could only see the others through the virtual world — but the others were people they actually worked with. Their shift boss was their actual shift boss. They were assigned tasks that they would be doing in real life. They had a radio in the virtual world for communication. The younger miners saw that it could be similar to a massive multiplayer online game they might play. After about 20 minutes inworld, the radio chatter indicated that they were fully immersed, cracking jokes like they do on the real radio, asking each other where others were, reporting safety hazards they found, and asking for direction on some tasks. There were about 30-35 people inworld together at the same time, not including the people logged in to run the show.
What they didn’t expect was that once deep in the virtual mine, an upset would occur at some point, 45 minutes to an hour into the session. Usually this was a fire in some random area of the mine. The actual training goal was to improve decision making following an accident that required the miners to evacuate or, if that wasn’t possible, to move quickly to a safe area with adequate ventilation, food and water. The real purpose of the exercise was to see how they handled the emergency. The underground tunnels filled with smoke and visibility got very poor. If they were near a fire, the noise was deafening. The light on their helmet bounced off the smoke and haze and the view could be deceptive; they could easily get lost or miss signs. Their avatar’s performance degraded; without oxygen, they were soon on the ground, unable to get up, with only minutes left to live. They had rebreathers that gave them about 30 minutes of air, but they had to decide when to put them on, and then pick an exit path, or a path that took only 30 minutes to reach a rescue chamber.
Before the exercise, all participants were briefed on the procedures and what parts of the mine were too far from the exits to reach safely. They were all briefed on the locations of rescue chambers and the reasons for going to them. Rescue chambers are crowded and unpleasant underground boxes for sustaining small groups of people with food, air and water until help can arrive. Understandably, people do not want to end up there and can easily get to one and find it is already full. It turned out that all the miners believed they knew how to get out and none believed they needed to go to a rescue chamber. This was not the case. During the exercise, many of the participants (i.e., their avatars) did not make it out alive. Some that did not, died because they misjudged how long their air would last and thought they had enough time to go back looking for others they could hear calling for help. It was heart-breaking to hear.
Everyone was dismayed by the results. The miners in particular felt traumatised, realising that about 30% of their colleagues would have died if this was a real-world event.
The next day the miners returned an hour early, at 7am, and took the exercise more seriously. Klein writes that the miners stopped complaining about the exercise, and ‘were determined to diagnose what had gone wrong, what was wrong with their approach, and how they needed to rethink their response.’
Every miner escaped on the second run. Those who had ‘survived’ the first run did so faster on the second run. Nobody died.
And here was the aftermath:
When the WTRI research team returned 8 months later for follow-up interviews, they found the effect had endured in the people who work underground. They reported that they look at the underground space very differently now. The first thing they do in an unfamiliar mine is imagine a problem and mentally simulate getting to safety. Some said they are more aware of hazards as well, things that might cause a problem such as a fire.
Note that such qualitative extraction was probably the result of a cognitive probe, similar to Klein’s ‘Critical Decision Method’.
Source: Training As if Your Life Depended On It
Maxx Appliances (2019)
In the late 2010s WTRI began focusing on scaling up the strategic rehearsal technology. ‘Maxx’ was part of a series of attempts to apply rehearsal simulation to more generic skills like project management, leadership, and inclusion. This was enabled by a game engine WTRI had licensed and built on. The basic idea was to have participants come in to run a company named ‘Maxx Inc’, and to practice agile project management skills during the development of a new product.
The Maxx “company” is richly represented; the website has an annual report, internal memos, financial performance reports and documents tellling its history. Challenges are realistic, very difficult, and replicate the kinds of events that put major efforts at risk. Events are slightly different for each team, with variation generated by a special Event Generator.
The team members log in from their own computers from anywhere, and meet in the Maxx world as a team, interacting to make Maxx Inc. successful. The story unfolds over a period of compressed “months”. The team works together in the Maxx world according to a convenient schedule. The team meets in the world with a facilitator at scheduled times and can get access the help desk with technical problems anytime. In the single player version, the participant manages a robotic team alone. (…)
In this study, there were 10 teams of 4 people each doing the activity together, and 31 people doing the exercise as individuals. As indicated, Maxx was designed so that when an individual or team performs well at the challenges facing the Maxx Company in the marketplace, they have developed judgement, mental simulation capability and stakeholder influencing capabilities of someone who has 3-4 years seasoning as a leader in an agile organization. The participants in the pilot were junior managers and relatively unseasoned in agile approaches or managing performance in volatile markets. In other words, they had not worked for organizations which require agile thinking in order to remain competitive.
The target performance that WTRI had built into the Maxx simulation was taken from a cognitive task analysis of skilled IBM project managers.
Participants were evaluated on the following scoring dimensions:
- The return on sales as a result of their business decisions. Their goal was to go from 5.5% Ros to 14% ROS or better with the deployment of the technology. For the owners, that would mean a net dividend of $20Million a year to over $120Million. For a small company trying compete in the IoT space against appliance giants, this is quite significant.
- Their judgments, behavior and way of managing people was scored using the capabilities associated with agile leadership and agile management. These are the “soft skill” scores. In some cases, there was overlap. For example, a poorly handled meeting could result in information needed for an informed business decision not being revealed.
I’m not entirely sure how the soft skill scores were calculated, and the paper doesn’t say. (Was this done through pre-scripted dialog options? But if so, how do you simulate team meetings? The paper does mention that product decisions are done using a ‘voting mechanism’ run under time pressure, which does appear to be more trackable. But anyway.)
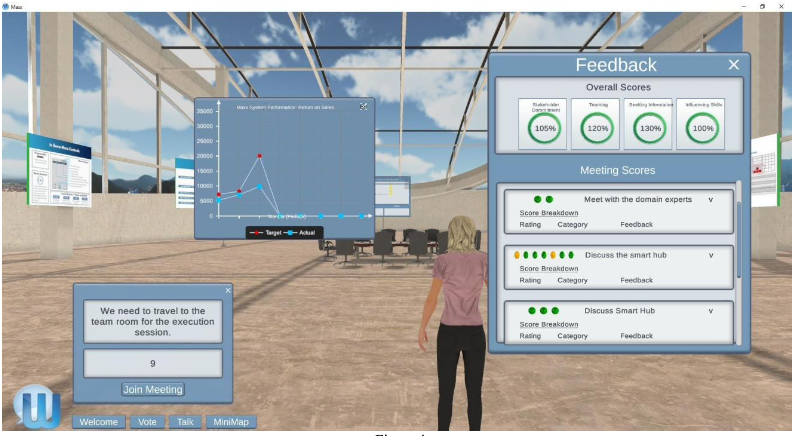
The exercise was run for two rounds within the space of two weeks, at no more than three hours per session.
One novel addition:
An interesting feature of Maxx is the Wendy app, a large “W” in the lower left the screen which, when clicked, accesses a number of handy tools, including the detailed dashboard, documents, a schedule and so on (Figure 4). We decided to have this large icon glow red, yellow or green depending on how the players were handling a given situation. The Icon, which is normally blue, would glow “red” if the participants were not handling the situation well, yellow if their handling was “okay” but not at the level of a seasoned expert, and “green” if it was handled the way a seasoned agile manager would conduct himself or herself.
We verified our scoring scheme for the “color glow” using experienced practicing agile managers, not agile coaches. Our feeling is that not all agile coaches have been managers and we wanted to have a scoring scheme road tested by those who had run companies. None of senior managers had access to the scoring scheme during the score testing. They simply did the exercise, having no insight into the reason for the colors. When all agreed on the “all green” set of behaviors we had our “upside” defined.
The results:
As we suspected, the teams of four did better on the first round, and improved markedly on the second round, achieving the desired ROS and averaging about 18% improvement in the quality of their agile judgements. All achieved the planned “upside”, showing judgement, as a team, associated with 3-4 years of experience on skills in the Agile Manifesto. The change was statistically significant despite the small number of teams (N= 10, df= 1, p<.007).
(…) As we expected, individuals very much struggled. Maxx is a difficult exercise and we got many calls for support – not with the technology, but with their anxiety. For one thing, it is harder to experiment and make mistakes without fear when you are doing something like this alone. However, once we got people over this hump, their statistically significant “jump” was higher than the teams' and higher than we expected, about 20% on average, with some individuals being much higher and with greater significance than the teams (N= 31, df= 1, p<.00016). All these individuals also met or exceeded the business benefits for Maxx.
Note that the differences in ‘scores’ are simply a measurement of the difference in decision performance collected during the first and second runs, across all participants. I’m not interested in the statistical significance so much as I am in the cognitive probes that they used to track a change in mental models. (The recent replication crises in psychology has made me naturally suspicious of p-values, though I can see why they did it — Maxx is meant to be run in an automated fashion, with no human facilitation, at scale).
Exploratory Mining Simulation (2021)
The most recent use of a novel strategic rehearsal was in late 2020, through a collaboration with mineral finding technology company CTAC.
This is the least developed application of their virtual world technology — and the results are only tentative in nature. To put it simply, DiBello and team were asked to create a simulation for a mining company that was interested in managing a portfolio of 11 smaller mines and deposits. This wasn’t really a strategic rehearsal in the training sense — the company wanted to use the simulation to explore the feasibility of running this particular portfolio as a workable business. (Historically, mines only made economic sense when run at scale; this was an attempt at derisking a business experiment with smaller mines using newer technology.)
As of press time, DiBello et al are still onboarding the mines and are not fully operational in either the virtual world or in real life. They write:
We wondered if the all the features collectively could be used to make it possible for companies to more efficiently manage a collection of small mines as one large mine, through a central virtual control room which tracks all the financial performance of each mine, and the portfolio as a whole, while immersive digital twins allowed for strategy formation and experimentation in each mine, as each one is acquired and integrated into the portfolio for maximum performance. The behavioral tracking and feedback could “onboard” each worker not only to the centralized technologies running the managing the mines, but also the portfolio’s business goals, so that everyone would understand how they fit into the larger picture.
The results from this engagement are probably still a few years away.
Features of a Strategic Rehearsal
To wrap up, let’s take a look at the main features of the Strategic Rehearsal, as explained by DiBello in her chapter in The Oxford Handbook of Expertise.
There are four critical components to the rehearsals that turned out to be important to accelerating expertise.
1. Time compression;
2. A rich unfolding future that has a pattern;
3. A non-negotiable goal with multiple ways available to achieve it; and
4. A rich history that is similar to the past the participants have experienced but not identical.
Participants run through the rehearsal twice, experiencing several cycles of failure and detailed feedback before they hit on a solution that will work. The first day of the rehearsal does not go well; when a major organisational breakdown is present in real life, participants invariably fall back on default behaviours and fail in a way that replicates their current problems in the real workplace. At the start of the second round of the rehearsal, participants are given feedback based on various performance metrics from the activities of the previous day. Detailed data are provided that show the ideal versus the actual performance. The participants discuss among themselves three issues: (1) what went wrong, (2) what we will do differently this time, and (3) how will we know it is working (emphasis added). On Day 2 they are given an opportunity to rework and develop novel solutions. As such, the solutions they devise on Day 2 often reflect workable strategies to bring back into the real workplace. Because various teams can coordinate across multiple functional areas with dynamic, real time feedback in a consequence-free environment, the rehearsal allows old systems to be discarded and new models emerge.
There are nuances, of course:
- Many of the Strategic Rehearsals depend on cognitive probes in order to surface the default mental models, as well as the degree of cognitive reorganisation that has occurred. (This is a fancy way of saying ‘cognitive task analysis’ — or ... ‘the set of tacit skill extraction techniques that emerged out of the Naturalistic Decision Making literature’). During her PhD years, DiBello published a paper on a quantitative cognitive probe approach by collecting data from ERP systems (DiBello, 1997) — though it’s likely that the technique has itself evolved. In the bus maintainers paper, for instance, she mentions that the probes were qualitative, and similar to Gary Klein’s Critical Decision Method; in the Maxx paper she writes that her team had built quantitative cognitive probes into the tasks tested within the Maxx virtual world.
- In my interview with her she mentioned that constructing a strategic rehearsal for a business context demands that they do an initial analysis of the business. That method is proprietary; it’s not likely to be published anytime soon.
As I’ve mentioned earlier, I’m not entirely sure how to put this to practice. But as an instantiation of the learning theories in Accelerated Expertise, I find the technique to be deeply compelling. I hope you’ve found this inspiring, or at least interesting.
We’ll talk about those learning theories soon.
Originally published , last updated .
This article is part of the Operations topic cluster, which belongs to the Business Expertise Triad. Read more from this topic here→